January 4th Big Model Daily Collection
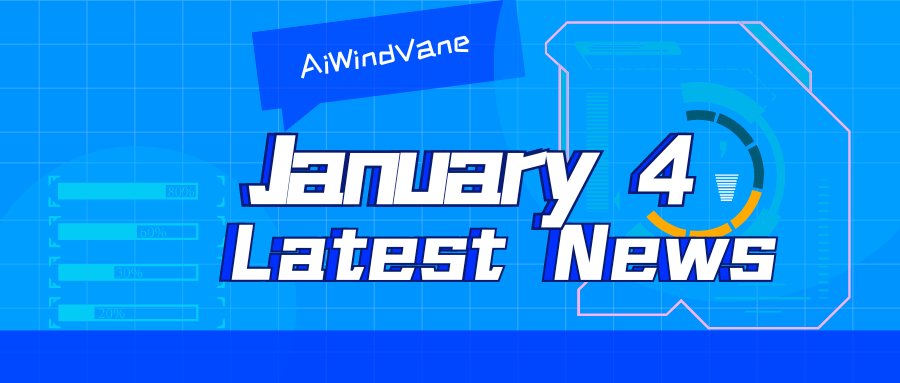
[January 4th Big Model Daily Collection] Let the robot sense your “Here you are”, the Tsinghua team uses millions of scenes to create universal human-machine handover; Li Feifei’s team’s new work: AI perspective eyes, see you clearly through obstacles, rendering and blocking the human body There is a new breakthrough
Let the robot sense your “Here you are”, the Tsinghua team uses millions of scenarios to create universal human-machine handover
Link: https://news.miracleplus.com/share_link/14990
Researchers from Tsinghua University’s Interdisciplinary Information Institute proposed the “GenH2R” framework to allow robots to learn generalizable vision-based human-to-robot handover policies. This generalizable strategy allows the robot to more reliably catch objects with diverse geometric shapes and complex motion trajectories from people’s hands, providing new possibilities for human-computer interaction.
Li Feifei’s team’s new work: AI see-through eyes can see you clearly through obstacles, and there is a new breakthrough in rendering and blocking the human body
Link: https://news.miracleplus.com/share_link/14991
AR/VR, movies, medical and other fields are widely using video to render human images. Since video acquisition from a monocular camera is relatively easy, rendering the human body from a monocular camera has been the main method of research. Methods such as Vid2Avatar, MonoHuman, and NeuMan have achieved impressive results. Despite having only one camera view, these methods accurately render the human body from new perspectives. Recently, Li Feifei, a well-known artificial intelligence professor, released new progress in 3D human rendering work on
Quickly sift through massive data and make informed decisions instantly, MIT, Princeton & Carnegie Mellon University teams use LLM for fusion research
Link: https://news.miracleplus.com/share_link/14992
Controllable nuclear fusion energy has the advantages of safety, cleanliness, and abundant fuel, and is one of the main options to solve mankind’s future energy problems. Perhaps the most promising nuclear fusion device is the Tokamak. Despite the promising prospects, important unanswered questions remain between human and economical tokamak power plants. Now, scientists from Princeton University, Carnegie Mellon University (CMU), and the Massachusetts Institute of Technology (MIT) have applied large-scale language models—the driving force behind tools like ChatGPT, Bard, and LLaMA—to help fusion researchers quickly Sift through incredible amounts of data to make smarter decisions instantly. The model allows users to identify previous experiments with similar characteristics, provide information about equipment control systems, and quickly return answers to questions about fusion reactors and plasma physics.
Nature sub-journal | Reverse design of nonlinear mechanical metamaterials through video denoising diffusion model
Link: https://news.miracleplus.com/share_link/14993
The reverse design of complex material properties has great potential in solving soft robotics, biomedical implants, and textile engineering. Although machine learning models provide such inverse mappings, they are often limited to linear target properties. Recently, researchers at the Federal Institute of Technology in Zurich (ETH Zurich) have demonstrated that a video diffusion generation model trained on full-field data of periodic random cellular structures can successfully predict and adjust them in large strain states in order to tailor nonlinear responses. Nonlinear deformation and stress response under conditions including buckling and contact. The key to success is to break away from the common strategy of directly learning mappings from properties to designs and extend the framework to intrinsically estimate expected deformation paths and full-field stress distributions, which is in good agreement with finite element simulations. This research therefore has the potential to simplify and accelerate the identification of materials with complex target properties.