January 8th Big Model Daily Collection
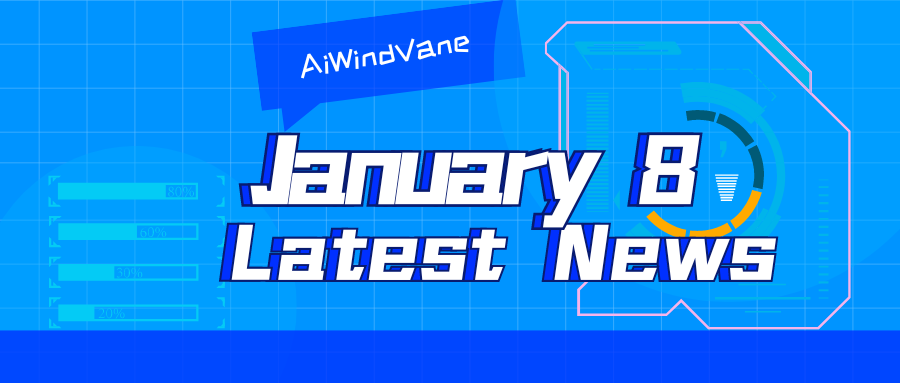
[January 8th Big Model Daily Collection] AI interpretation video comes at your mouth? This “illusion” problem is solved with Vista-LLaMA; four lines of code triple the context of a large model, applicable to alpaca Mistral; low energy consumption and high speed, a new method from EPFL and Microsoft research teams: deep physics neural network Backpropagation-free training; Microsoft executive Dee Templeton joins OpenAI board of directors
AI interprets videos just by opening your mouth? This “illusion” problem is solved with Vista-LLaMA
Link: https://news.miracleplus.com/share_link/15242
In recent years, large-scale language models such as GPT, GLM, and LLaMA have made significant progress in the field of natural language processing, and can understand and generate complex text content based on deep learning technology. However, extending these capabilities to the field of video content understanding is a brand new challenge – videos not only contain rich and varied visual information, but also involve dynamic changes in time series, which makes it more difficult for large language models to extract information from videos. For complexity. Faced with this challenge, Bytedance and Zhejiang University proposed Vista-LLaMA, a multi-modal large language model that can output reliable video descriptions. Specifically designed for the complexity of video content, Vista-LLaMA can effectively convert video frames into accurate language descriptions, thereby greatly improving the quality of video content analysis and generation.
Say goodbye to labeling one by one, use one prompt to achieve batch image segmentation, efficient and accurate
Link: https://news.miracleplus.com/share_link/15243
The proposal of Segment Anything Model (SAM) has attracted great attention in the field of image segmentation, and its excellent generalization performance has aroused widespread interest. However, despite this, SAM still faces an unavoidable problem: in order for SAM to accurately segment the location of the target object, each picture needs to be manually provided with a unique visual cue. Some current methods, such as SEEM and AV-SAM, guide the model to better understand what objects are to be segmented by providing input information from more modalities. Researchers from Queen Mary, University of London have proposed a training-free segmentation method called GenSAM, which can effectively segment all unlabeled samples under the task while only providing a common text prompt for the task.
Four lines of code triple the context of a large model, applicable to alpaca Mistral
Link: https://news.miracleplus.com/share_link/15267
No fine-tuning is required, just four lines of code can dramatically increase the window length of a large model, up to 3 times! Moreover, it is “plug and play” and can theoretically be adapted to any large model. It has been successfully tested on Mistral and Llama2. With this technology, a large model (LargeLM) can be transformed into a LongLM. Recently, Chinese scholars from Texas A&M University and other institutions released a new large model window expansion method SelfExtended (SE for short). On Mistral, the researchers randomly inserted 5-digit numbers into the 24k-length text for the model to search. After SE processing, the results showed an all-green (passed) test result.
De novo peptide sequencing with over 40% sensitivity and 90% accuracy, a deep learning-driven tandem mass spectrometry analysis method
Link: https://news.miracleplus.com/share_link/15268
Unlike DNA and RNA, proteins lack accurate and high-throughput sequencing methods, which hinders the utility of proteomics in applications where sequence is unknown, including variant calling, novel epitope identification, and metaproteomics. Researchers at Technische Universität München (TUM) in Germany have introduced Spectralis, a de novo peptide sequencing method for tandem mass spectrometry analysis. Spectralis leverages several innovations, including convolutional neural network layers that connect spectral peaks spaced by amino acid mass, proposing fragment ion series classification as a key task for de novo peptide sequencing, and peptide map confidence scoring. For real spectra provided by database searches, Spectralis achieves a sensitivity of over 40% and an accuracy of up to 90%, almost twice the sensitivity of current SOTA. Application to unidentified spectra confirms its superiority and demonstrates its suitability for variant calling.
Low energy consumption and high speed, a new method from EPFL and Microsoft research teams: backpropagation-free training of deep physics neural networks
Link: https://news.miracleplus.com/share_link/15269
With recent developments in large-scale deep neural networks (NN) and other artificial intelligence (AI) applications, there are growing concerns about the energy consumption required to train and operate them. Physical neural networks can be a solution to this problem, but direct hardware implementation of traditional algorithms faces multiple difficulties. Training neural networks using the traditional backpropagation algorithm comes with several challenges, such as lack of scalability, complexity of operations during training, and reliance on digital training models. A collaborative team including École Polytechnique Fédérale de Lausanne (EPFL) and Microsoft Research has proposed a simple deep neural network architecture enhanced by the Physical Local Learning (PhyLL) algorithm that can Supervise and unsupervised training of deep physics neural networks without detailed knowledge of the properties of the nonlinear physics layer. Using this approach, the researchers trained a variety of wave-based physics neural networks in experiments on vowel and image classification and demonstrated the generalizability of the approach.
The speed of multi-round dialogue reasoning is increased by 46%, and the open source solution breaks the length limit of LLM multi-round dialogue
Link: https://news.miracleplus.com/share_link/15244
In the world of large language models (LLMs), handling multiple turns of dialogue has always been a challenge. StreamingLLM, recently launched by MIT Guangxuan Xiao and others, can achieve streaming input of a total of 4 million tokens in multiple rounds of dialogue without sacrificing inference speed and generation effects, increasing the inference speed by 22.2 times. However, StreamingLLM is implemented using native PyTorch, and there is still room for optimization for low-cost, low-latency, high-throughput and other requirements for multi-round dialogue reasoning scenarios. The Colossal-AI team has open sourced SwiftInfer and implemented StreamingLLM based on TensorRT, which can further improve large model reasoning performance by 46% and provide an efficient and reliable implementation solution for multi-round dialogue reasoning.
Microsoft executive Dee Templeton joins OpenAI board of directors
Link: https://news.miracleplus.com/share_link/15270
On January 6, Bloomberg reported that Microsoft executive Dee Templeton joined the OpenAI board of directors as a non-voting observer. Currently, OpenAI has a total of 4 directors, including former Salesforce co-CEO Bret Taylor (as chairman), former U.S. Treasury Secretary Larry Summers, Adam D’Angelo, co-founder of Quora, the world’s largest knowledge question and answer community, and newly joined Dee. This is also the first time Microsoft has appointed people to the OpenAI board of directors since it invested US$1 billion in OpenAI on July 22, 2019.