June 4th Big Model Daily Collection
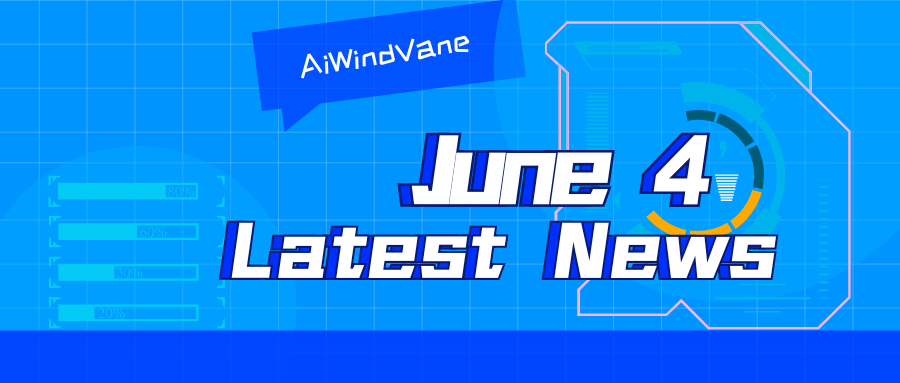
[June 4th Big Model Daily Collection] News: Fight Transformer again! Mamba 2 led by the original author is here, and the new architecture training efficiency is greatly improved; Multimodal model learns to play poker: performance exceeds GPT-4v, and the new reinforcement learning framework is the key
Fight Transformer again! Mamba 2 led by the original author is here, and the new architecture training efficiency is greatly improved
Link: https://news.miracleplus.com/share_link/28947
Since it was proposed in 2017, Transformer has become the mainstream architecture of AI big models and has always been in the C position in language modeling. However, as the model scale expands and the sequences that need to be processed continue to grow, the limitations of Transformer are gradually becoming more prominent. One obvious flaw is that the amount of computation of the self-attention mechanism in the Transformer model will increase quadratically with the increase of context length. A few months ago, the emergence of Mamba broke this situation, and it can achieve linear expansion with the increase of context length. With the release of Mamba, these state-space models (SSMs) have achieved comparable or even superior performance to Transformers at small and medium scales. Mamba was written by only two authors, Albert Gu, Assistant Professor of Machine Learning at Carnegie Mellon University, and Tri Dao, Chief Scientist at Together.AI and Assistant Professor of Computer Science at Princeton University. The community responded enthusiastically to Mamba in the period after its release. Unfortunately, Mamba’s paper was rejected by ICLR, which surprised many researchers. Just six months later, the original authors led the release of the more powerful Mamba 2.
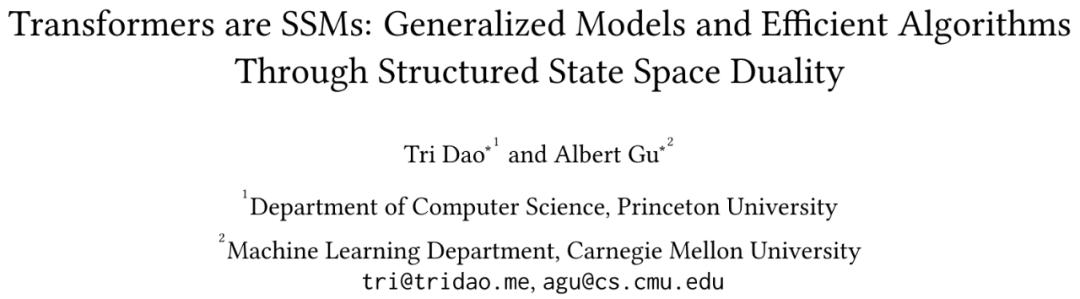
Multimodal models learn to play poker: Outperforming GPT-4v, a new reinforcement learning framework is the key
Link: https://news.miracleplus.com/share_link/28948
Using only reinforcement learning for fine-tuning, without human feedback, large multimodal models can learn to make decisions! The model obtained by this method has learned tasks such as playing poker by looking at pictures and calculating “12 points”, and its performance even surpasses GPT-4v. This is the latest fine-tuning method proposed by universities such as UC Berkeley, and the research lineup is also quite luxurious:
* LeCun, one of the three giants of the Turing Award, chief AI scientist of Meta, and professor of New York University
* Sergry Levine, a UC Berkeley expert and member of the ALOHA team
* Xie Saining, the author of ResNeXt() and DiT, the basic technology of Sora
* Ma Yi, dean of the School of Data Science at the University of Hong Kong and professor of UC Berkeley
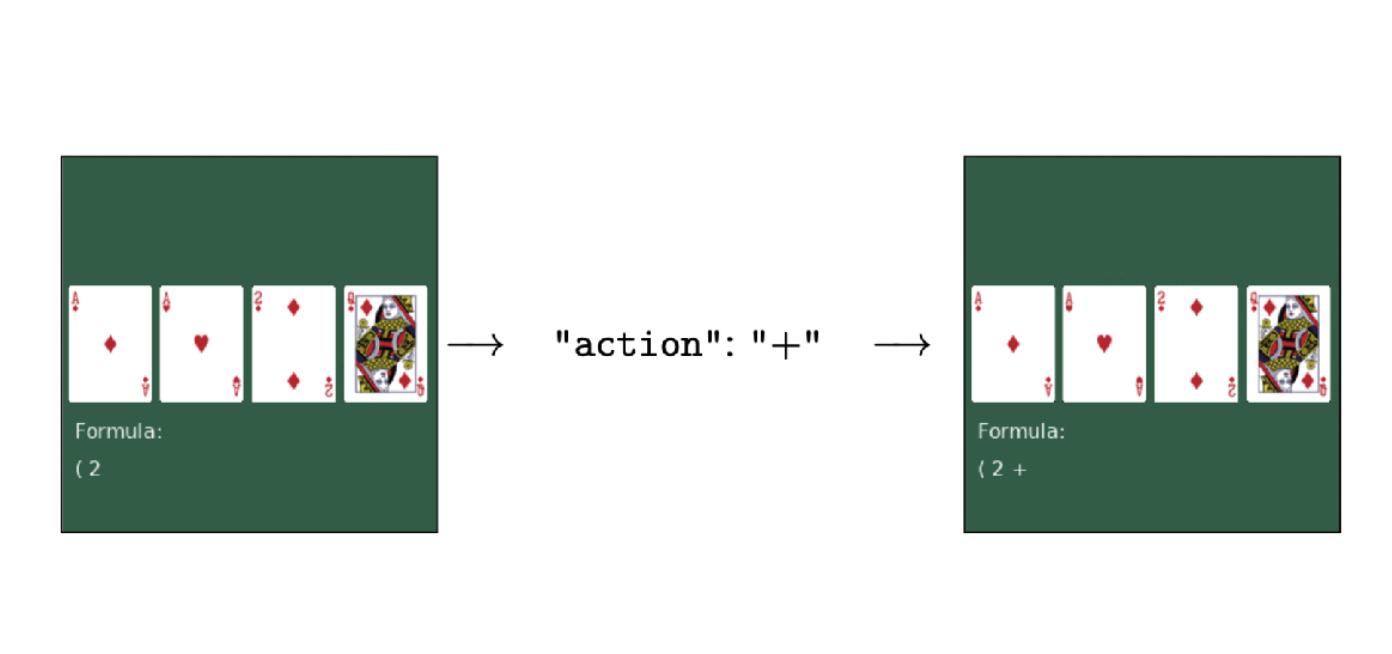
Oculus founder Palmer Luckey claims that he is developing a new head-mounted display device
Link: https://news.miracleplus.com/share_link/28949
AWE USA 2024 will be held in Los Angeles, California, USA from June 18 to June 20, and Oculus founder Palmer Luckey recently announced that he plans to officially announce the VR head-mounted display device he is researching at this event.
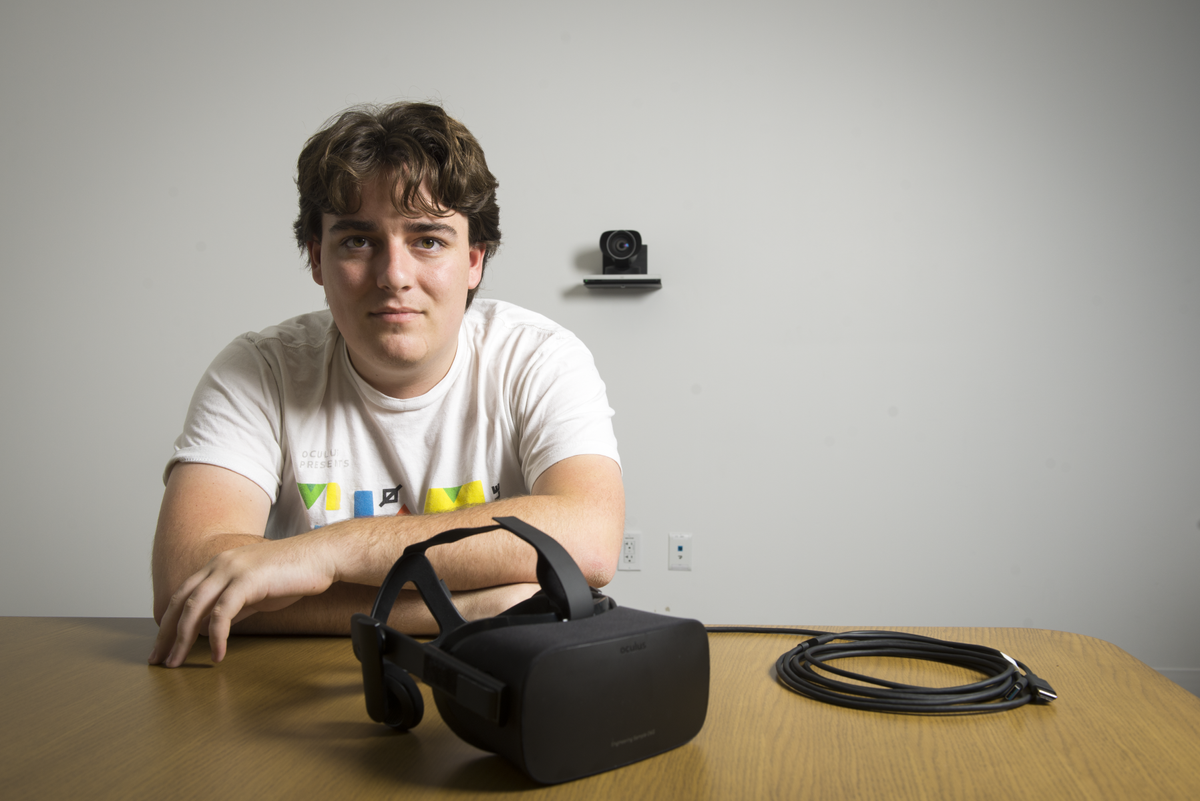
Karpathy likes this report, which teaches you how to create high-quality web datasets with LLaMa 3
Link: https://news.miracleplus.com/share_link/28950
It is well known that building high-quality web-scale datasets is very important for high-performance large language models such as Llama3, GPT-4 or Mixtral. However, even the pre-training datasets of the most advanced open source LLMs are not public, and little is known about their creation process. Recently, AI expert Andrej Karpathy recommended a work called FineWeb-Edu.
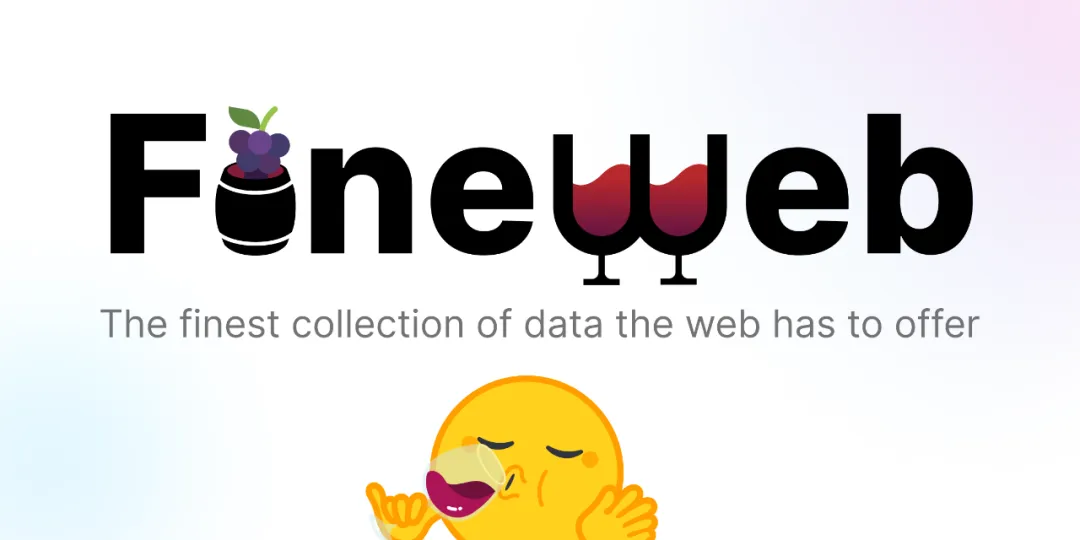
Single 4090 reasonable, 200 billion sparse large model “Skywork MoE” open source
Link: https://news.miracleplus.com/share_link/28951
In the wave of large models, training and deploying state-of-the-art dense LLMs poses huge challenges in terms of computing requirements and associated costs, especially at the scale of tens or hundreds of billions of parameters. To address these challenges, sparse models, such as mixture of experts (MoE), have become increasingly important. These models provide a more economically viable alternative by allocating computations to various specialized sub-models or “experts”, with the potential to reach or even exceed the performance of dense models with extremely low resource requirements. On June 3, another important news came from the field of open source large models: Kunlun Wanwei announced the open source of the 200 billion sparse large model Skywork-MoE, which significantly reduced the cost of inference while maintaining strong performance. Skywork-MoE is based on the Skywork-13B model intermediate checkpoint previously open-sourced by Kunlun Wanwei. It is the first open-source 100 billion MoE large model that fully applies and implements the MoE Upcycling technology, and is also the first open-source 100 billion MoE large model that supports reasoning with a single 4090 server. What attracts more attention from the large model community is that the model weights and technical reports of Skywork-MoE are completely open-source and free for commercial use without application.
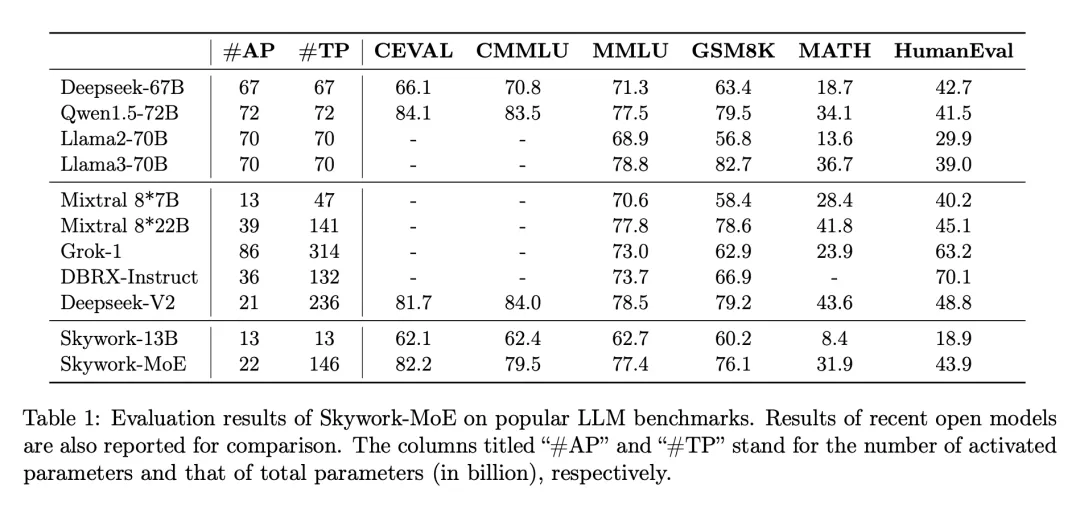
Copyright protection of AI training data: tragedy of the commons or prosperity of cooperation?
Link: https://news.miracleplus.com/share_link/28952
The case of GPT-4o’s built-in voice imitating “Black Widow” has caused a sensation, although it has ended in stages with OpenAI’s announcement to suspend the use of the voice of “SKY” suspected to be Black Widow’s voice and denying that it has infringed the voice. However, the topic of “even AI must protect human copyright” has become popular, further stimulating people’s anxiety about whether AI is controllable, a modern myth. Recently, Princeton University, Columbia University, Harvard University and the University of Pennsylvania jointly launched a new solution for copyright protection of generative AI, entitled “An Economic Solution to Copyright Challenges of Generative AI”.
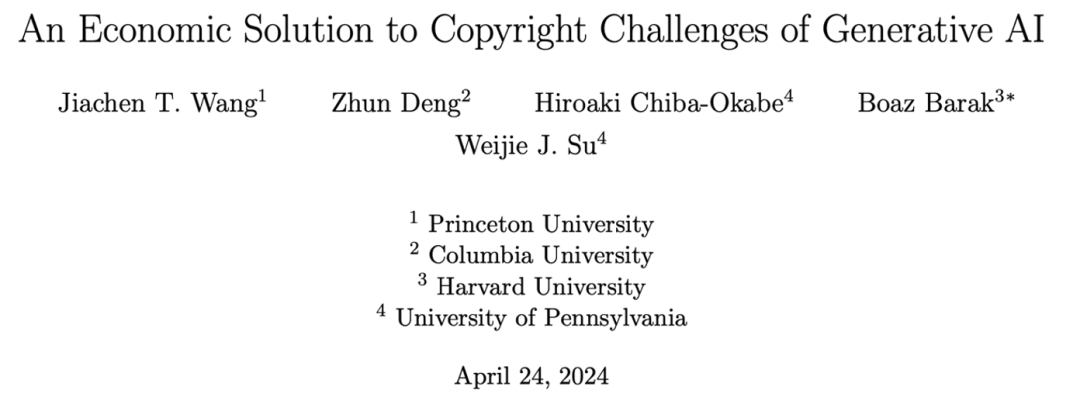
Wall Street takes stock of the global chip war! The market size will reach 1 trillion US dollars
Link: https://news.miracleplus.com/share_link/28953
According to the Wall Street Journal, as the global chip war escalates, the size of the chip industry is expected to double to 1 trillion US dollars by the end of this century. Governments are stepping up efforts to promote domestic chip production, which powers industries such as automobiles, electronics and artificial intelligence. Companies around the world are racing to join the trend. Global semiconductor revenue is expected to exceed $1 trillion by the end of the decade, according to International Business Strategies, a chip industry consulting firm. Greater domestic production capacity could diversify the highly specialized semiconductor supply chain, which has strengths in certain process areas in certain regions and weaknesses in others. For example, U.S. companies lead in many areas of chip design, while companies in Taiwan, South Korea and mainland China dominate the later stages of production and assembly.
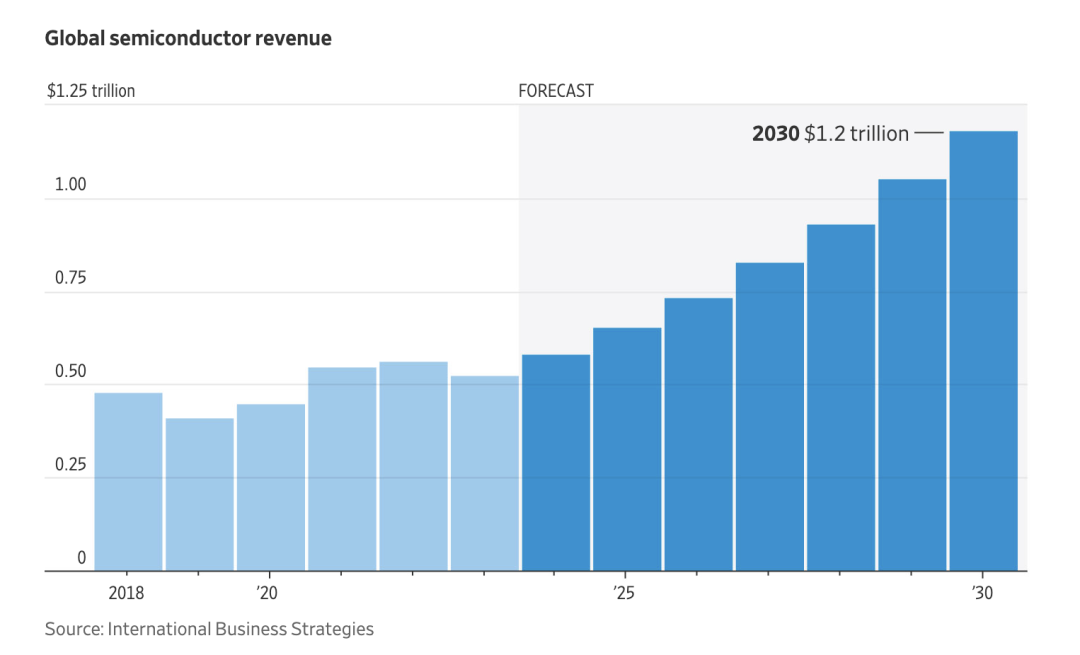