Big Model Daily (June 13)
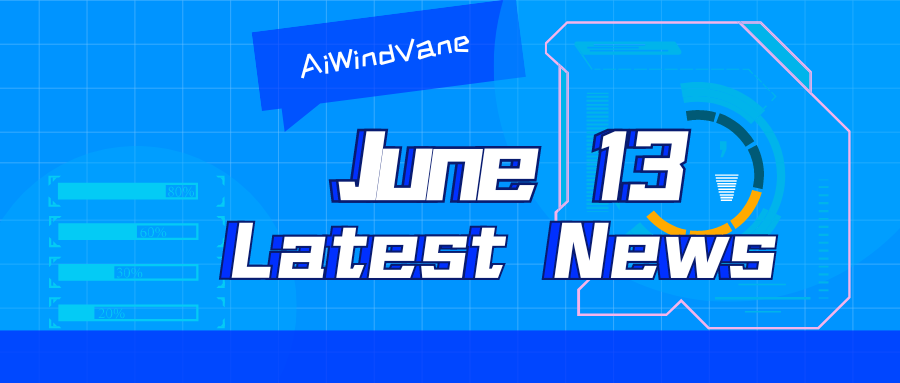
[Big Model Daily (June 13)] Information: ① IDC latest report, 11 big model manufacturers compete in 7 dimensions, who is the only one with full excellence? ② Stability Al open source Stable Diffusion 3 Medium text and image model; Paper: ① What if we use LLaMA-3 to add descriptions of billions of online pictures again? Investment and financing: Black Semiconductor raises $274 million to promote the development of European chips
IDC latest report, 11 big model manufacturers compete in 7 dimensions, who is the only one with full excellence?
https://news.miracleplus.com/share_link/29917
If the test questions are too simple, even a poor student can get 100 points. In the AI circle, what kind of “test paper” should we take to test the true level of the big model that has always been in the C position of traffic? Is it the college entrance examination question? Of course not! Some people also think that whoever ranks first on various Benchmark lists is the strongest. In fact, this is not the case. Sometimes, the more “authoritative” the list is, the easier it is to be strategically brushed. Therefore, the “strength” of a model cannot just be ranked first on a certain Benchmark, but must be strong in multiple dimensions. Recently, the world’s leading International Data Corporation (IDC) released the latest large model test report “Evaluation of Mainstream Products in China’s Large Model Market, 2024”, which tested 16 mainstream products in the market from 11 large model manufacturers from 7 dimensions from basic capabilities to application capabilities. The report shows that Baidu Wenxin’s overall competitiveness in the big model is at the leading level, and its product capabilities are in the first echelon. It is the only company that is an advantageous manufacturer in all 7 dimensions. Wenxin Yiyan and Wenxin Yige have leading advantages in 7 dimensions, including basic capabilities such as question and answer understanding, reasoning, creative expression, mathematics, and code, and application capabilities such as toC general scenarios and toB specific industries. Among other evaluated manufacturers, Alibaba won 6 advantageous dimensions, and OpenAI GPT-4 and SenseTime won 5 respectively.
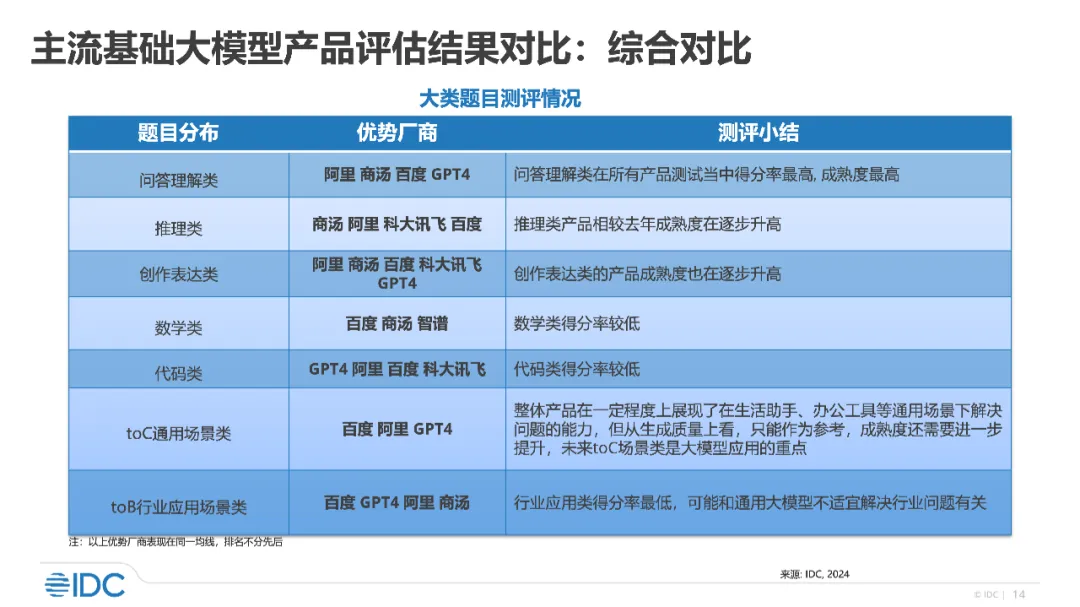
Stability AI open-sources Stable Diffusion 3 Medium text-to-image model
https://news.miracleplus.com/share_link/29833
On the evening of June 12, artificial intelligence startup Stability AI announced the official open-source release of its latest text-to-image generation model, Stable Diffusion 3 Medium (SD3 Medium). Stable Diffusion 3 Medium contains 2 billion parameters and is StabilityAI’s most advanced text-to-image open model to date. Its smaller VRAM footprint is designed to make it more suitable for running on consumer-grade GPUs as well as enterprise-grade GPUs.
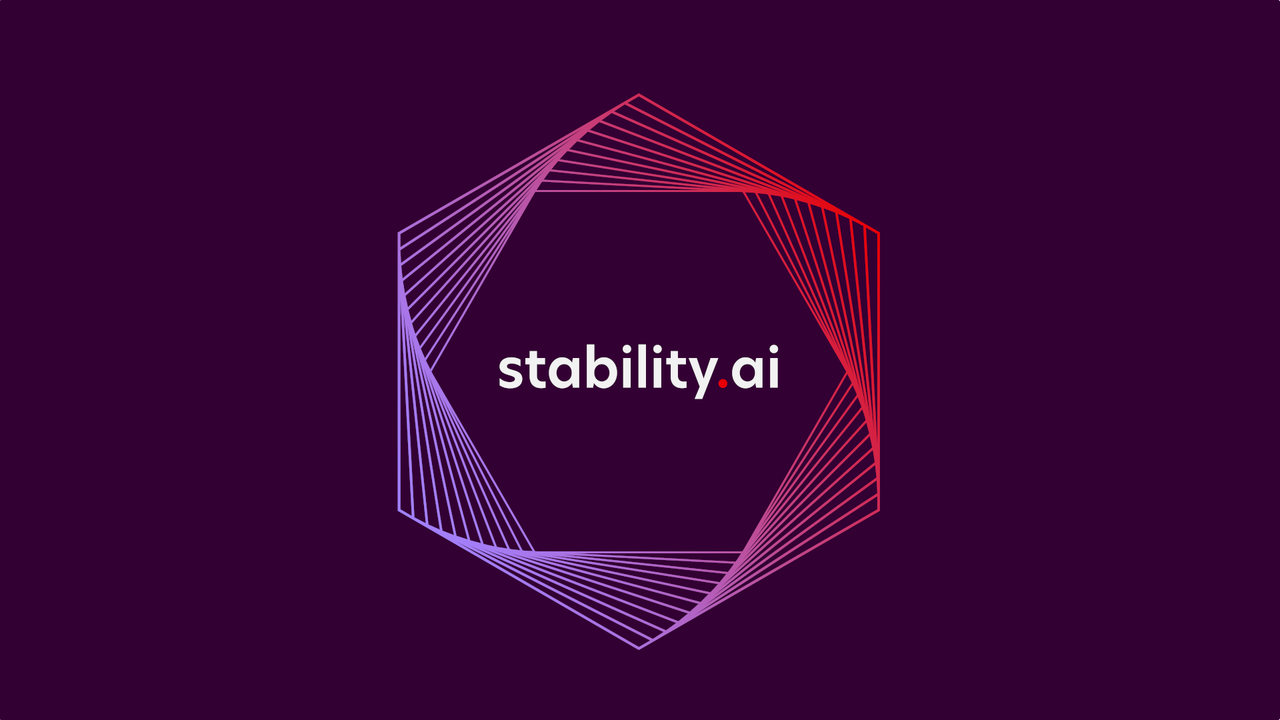
Another Sora-level player is here to blow up the streets!
https://news.miracleplus.com/share_link/29920
If Sora is not open for use, it will really be stolen! Today, San Francisco startup Luma AI (played a trump card and launched a new generation of AI video generation model Dream Machine. It is free for everyone.
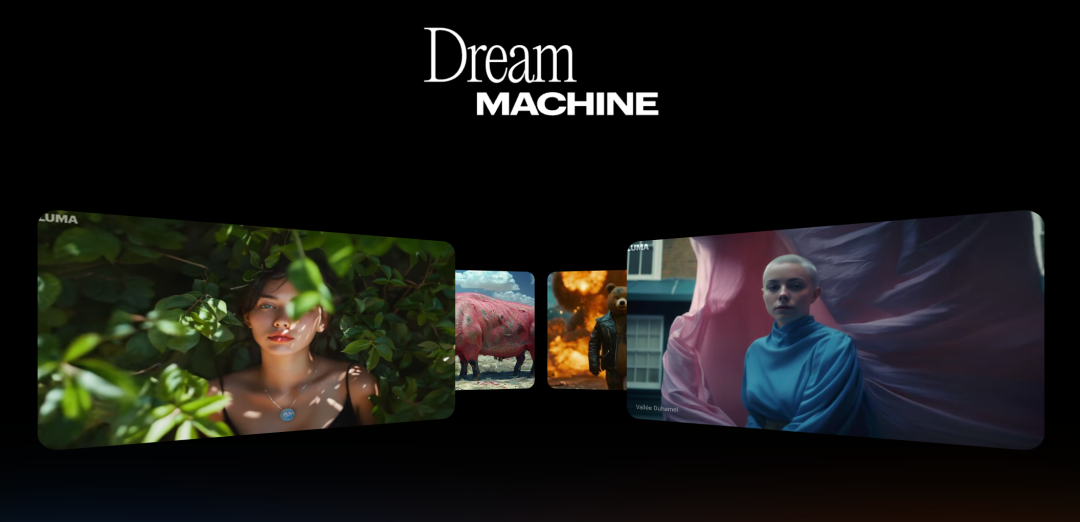
Ten thousand words review of efficient reasoning of large models: Wuwen Xinqiong, Tsinghua University and Shanghai Jiaotong University’s latest joint research comprehensively analyzes the reasoning optimization of large models
https://news.miracleplus.com/share_link/29921
In recent years, large language models (LLMs) have received widespread attention from academia and industry. Thanks to their outstanding performance in various language generation tasks, large language models have promoted the development of various artificial intelligence applications (such as ChatGPT, Copilot, etc.). However, the implementation of large language models is limited by their large reasoning overhead, which brings huge challenges to deployment resources, user experience, and economic costs. For example, to deploy and reason the LLaMA-2-70B model containing 70 billion parameters, at least 6 RTX 3090Ti graphics cards or 2 NVIDIA A100 graphics card. Taking the model deployed on the A100 graphics card as an example, it takes more than 50 seconds for the model to generate a 512-length token sequence. Many research works are dedicated to designing technologies to optimize the inference overhead of large language models. Optimizing the model’s inference latency, throughput, power consumption, and storage indicators has become an important goal of many studies. In order to have a more comprehensive and systematic understanding of these optimization technologies, and to provide advice and guidance for the deployment practice and future research of large language models, a research team from the Department of Electronic Engineering of Tsinghua University, Wuwen Xinqiong, and Shanghai Jiao Tong University conducted a comprehensive survey and compilation of efficient inference technologies for large language models. In “A Survey on Efficient Inference for Large Language Models” (LLM for short), the team conducted a comprehensive survey and compilation of efficient inference technologies for large language models. This 10,000-word long review of the field-related work is divided into three optimization levels (i.e., data level, model level, and system level), and introduces and summarizes the relevant technical work level by level. In addition, this work also analyzes the root causes of the inefficiency of large language model reasoning, and based on the review of the current existing work, it deeply explores the scenarios, challenges, and routes that should be paid attention to in the field of efficient reasoning in the future, providing researchers with feasible future research directions.
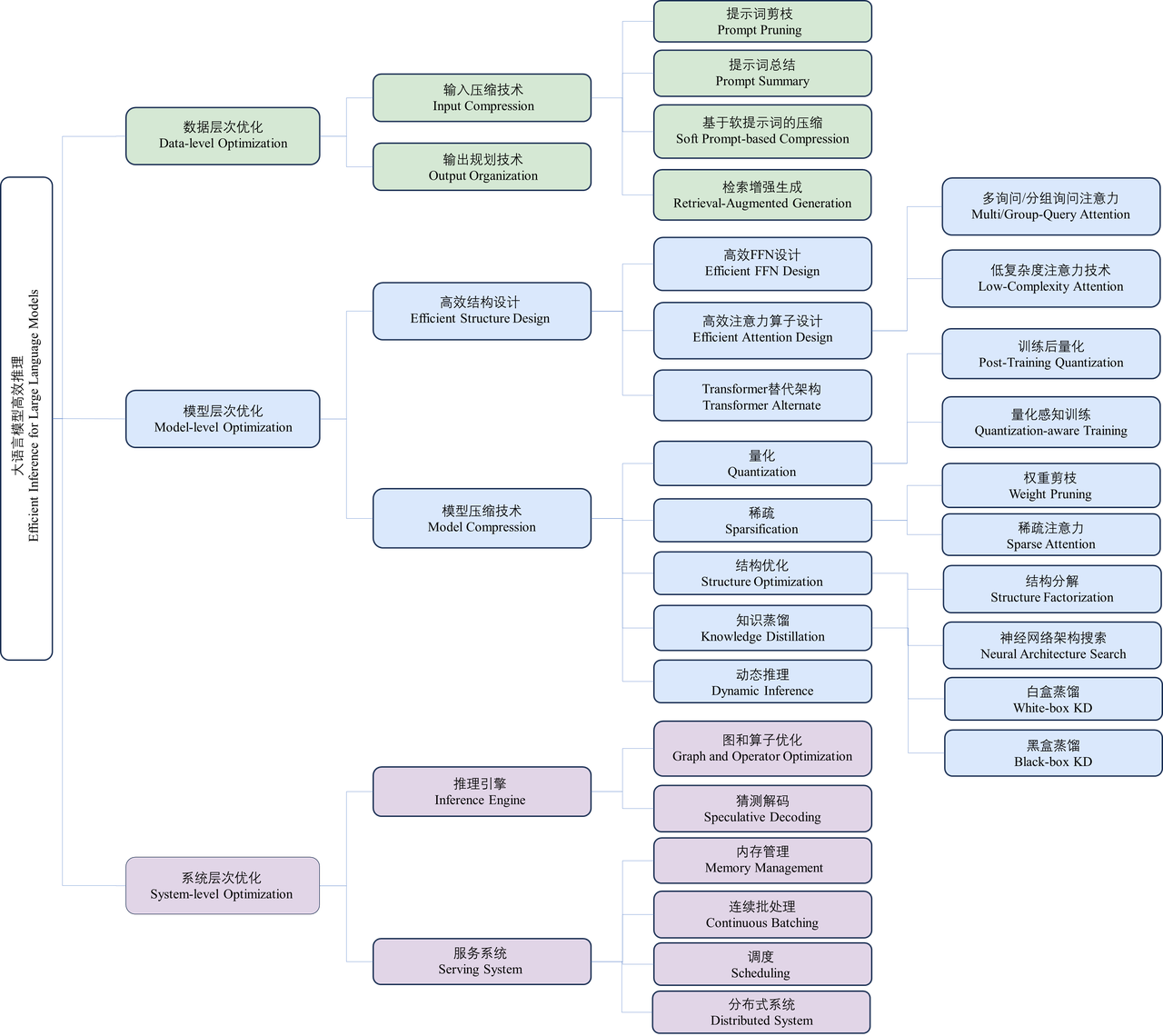
“AI+Physical Prior Knowledge”, Zhejiang University and Chinese Academy of Sciences’ universal protein-ligand interaction scoring method published in Nature sub-journal
https://news.miracleplus.com/share_link/29922
Proteins are like precision locks in the body, and drug molecules are keys. Only perfectly matched keys can unlock the door to treatment. Scientists have been looking for efficient ways to predict the matching degree between these “keys” and “locks”, that is, protein-ligand interactions. However, traditional data-driven methods often fall into “rote learning”, memorizing ligand and protein training data instead of truly learning the interactions between them. Recently, a research team from Zhejiang University and the Chinese Academy of Sciences proposed a new scoring method called EquiScore, which uses heterogeneous graph neural networks to integrate physical prior knowledge and characterize protein-ligand interactions in equivariant geometric space. EquiScore is trained on a new dataset that is constructed using multiple data augmentation strategies and strict redundancy elimination schemes. On two large external test sets, EquiScore consistently ranks among the top compared to 21 other methods. When EquiScore is used with different docking methods, it can effectively enhance the screening capabilities of these docking methods. EquiScore also performs well in a series of activity ranking tasks for structural analogs, indicating its potential to guide the optimization of lead compounds. Finally, different levels of interpretability of EquiScore were studied, which may provide more insights into structure-based drug design.
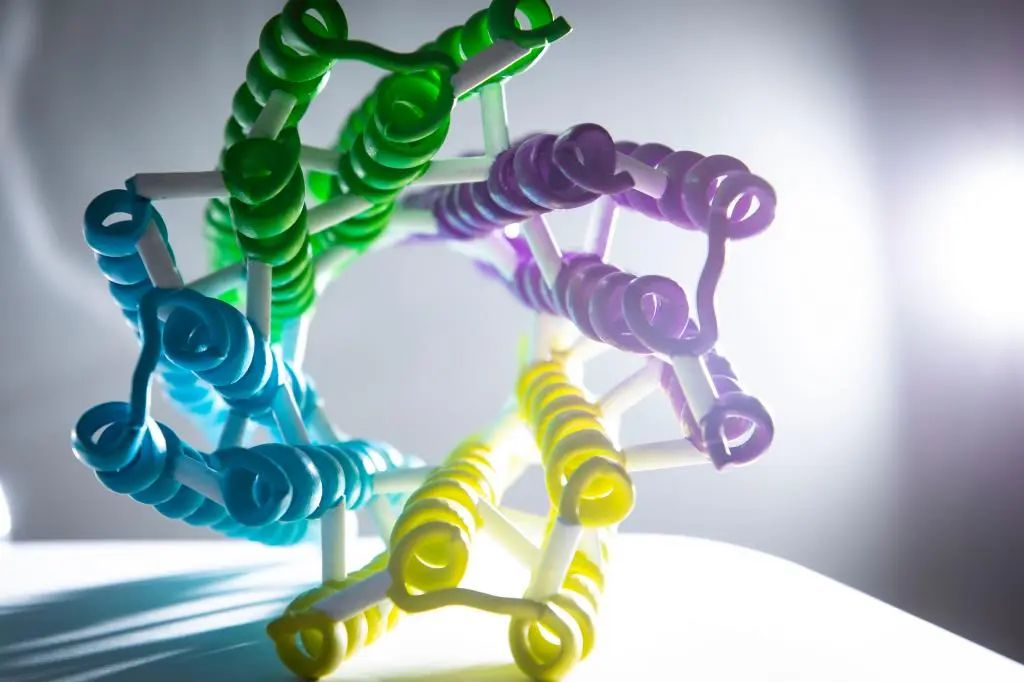