Collection of big model daily reports from October 21st to October 22nd
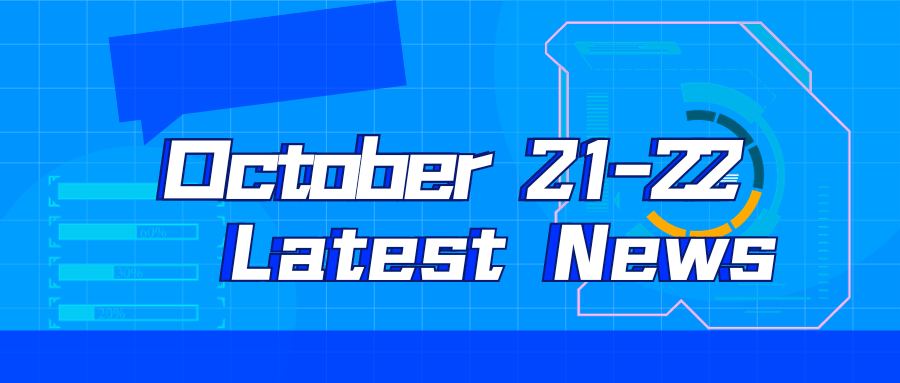
[Collection of big model daily reports from October 21st to October 22nd] The industry “black talk” between ChatGPT and DALL·E 3 was discovered; 22 billion transistors, IBM machine learning dedicated processor NorthPole, energy efficiency increased by 25 times; Musk’s first xAI research results released! Co-authored by founding member Yang Ge and Yao class alumni
The industry “black talk” between ChatGPT and DALL·E 3 was discovered
Link: https://news.miracleplus.com/share_link/11082
At the end of last month, OpenAI released the latest image generator DALL·E 3. In addition to the explosive generation effects, the biggest highlight is its integration with ChatGPT. DALL·E 3 is built on ChatGPT, using ChatGPT to create, extend and optimize prompts. This way, the user doesn’t need to spend too much time on the prompt. As users continued to test the functionality of the DALL·E 3 application, someone began to notice some very interesting bugs that revealed internal prompts shared between DALL·E 3 and ChatGPT. Curiously, the instructions included commands written in all caps for emphasis, demonstrating the possibility of human-like communication skills between AIs.
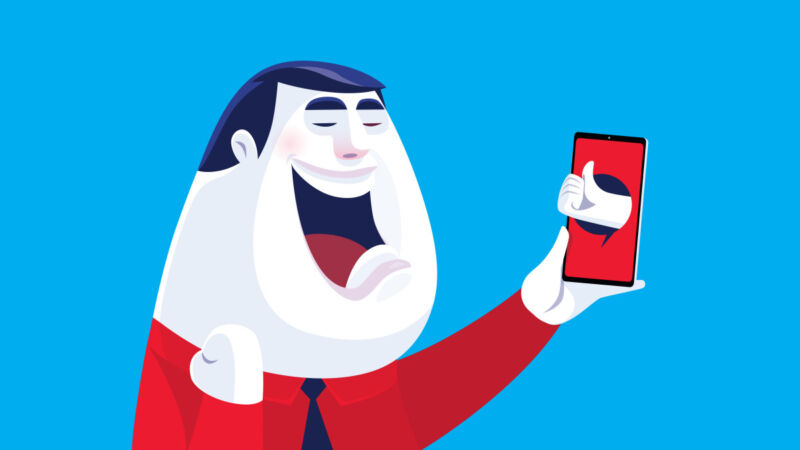
With GPT-4, the robot has learned how to spin pens and plate walnuts.
Link: https://news.miracleplus.com/share_link/11083
In terms of learning, GPT-4 is a great student. After digesting a large amount of human data, it has mastered various knowledge and can even inspire mathematician Terence Tao during chats. At the same time, it has also become an excellent teacher, not only teaching book knowledge, but also teaching the robot to turn pens. The robot, called Eureka, is the result of research from Nvidia, the University of Pennsylvania, Caltech, and the University of Texas at Austin. This research combines research on large language models and reinforcement learning: GPT-4 is used to refine the reward function, and reinforcement learning is used to train robot controllers. With the ability to write code in GPT-4, Eureka has excellent reward function design capabilities. Its independently generated rewards are better than those of human experts in 83% of tasks. This ability allows robots to complete many tasks that were not easy to complete before, such as turning pens, opening drawers and cabinets, throwing and catching balls, dribbling, and operating scissors. However, this is all done in a virtual environment for the time being.
The commenting ability is stronger than GPT-4, and the large model Auto-J is submitted to the open source 13B evaluation
Link: https://news.miracleplus.com/share_link/11084
With the rapid development of generative artificial intelligence technology, ensuring the alignment of large models with human values (intentions) has become an important challenge in the industry. Although model alignment is crucial, current evaluation methods often have limitations, which often leaves developers confused: How well aligned are large models? This not only restricts the further development of alignment technology, but also raises public concerns about the reliability of the technology. To this end, the Generative Artificial Intelligence Laboratory of Shanghai Jiao Tong University responded quickly and launched a new value alignment assessment tool: Auto-J, aiming to provide the industry and the public with a more transparent and accurate model value alignment assessment.
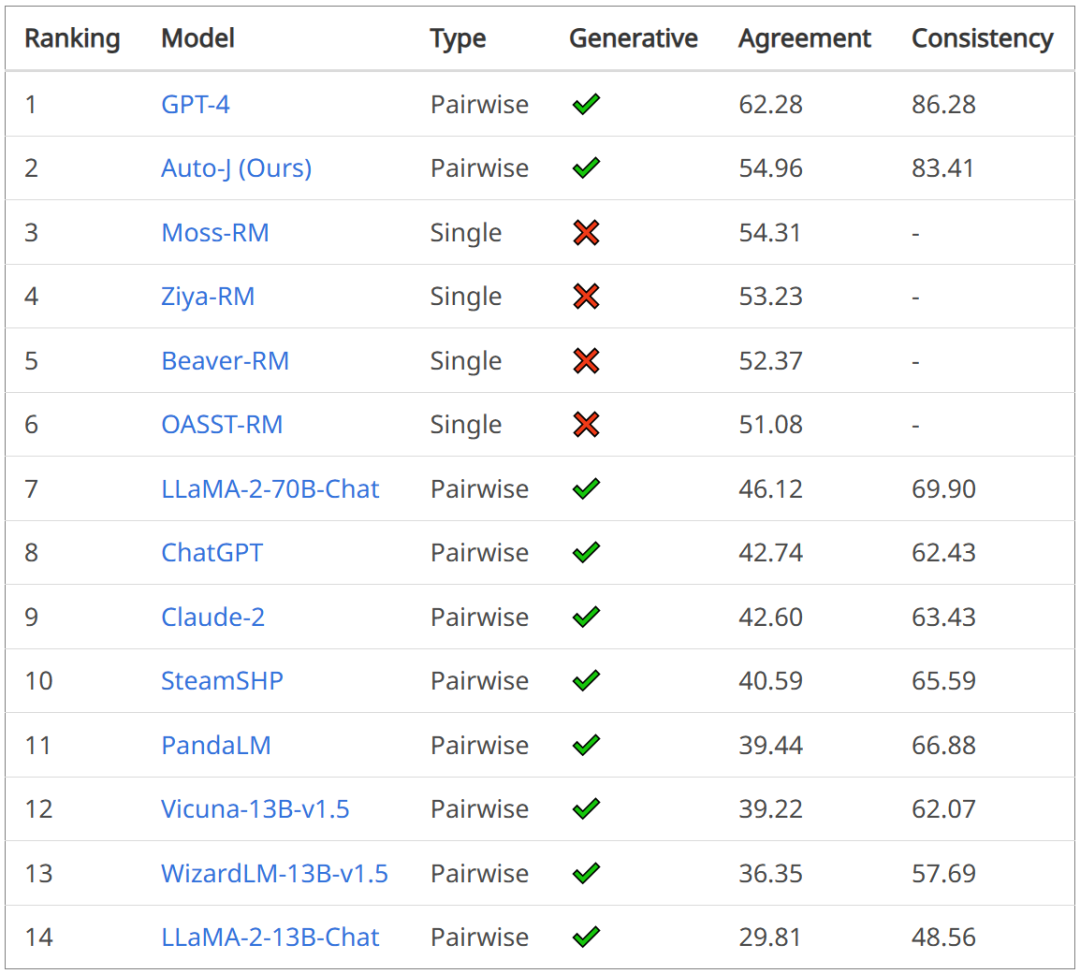
With nearly half the parameters, the performance is close to Google Minerva. Another large mathematical model is open source.
Link: https://news.miracleplus.com/share_link/11085
Researchers from Princeton University, EleutherAI and others have trained a domain-specific language model to solve mathematical problems. They believe that: first, solving mathematical problems requires pattern matching with a large amount of professional prior knowledge, so it is an ideal environment for domain adaptability training; second, mathematical reasoning itself is the core task of AI; finally, the ability to perform strong mathematical reasoning Language models are the upstream of many research topics, such as reward modeling, inference reinforcement learning, and algorithmic reasoning. Therefore, they propose a method to adapt language models to mathematics through continuous pre-training of Proof-Pile-2. Proof-Pile-2 is a mix of mathematics-related text and code. Applying this approach to Code Llama results in LLEMMA: a base language model for 7B and 34B, with greatly improved mathematical capabilities.
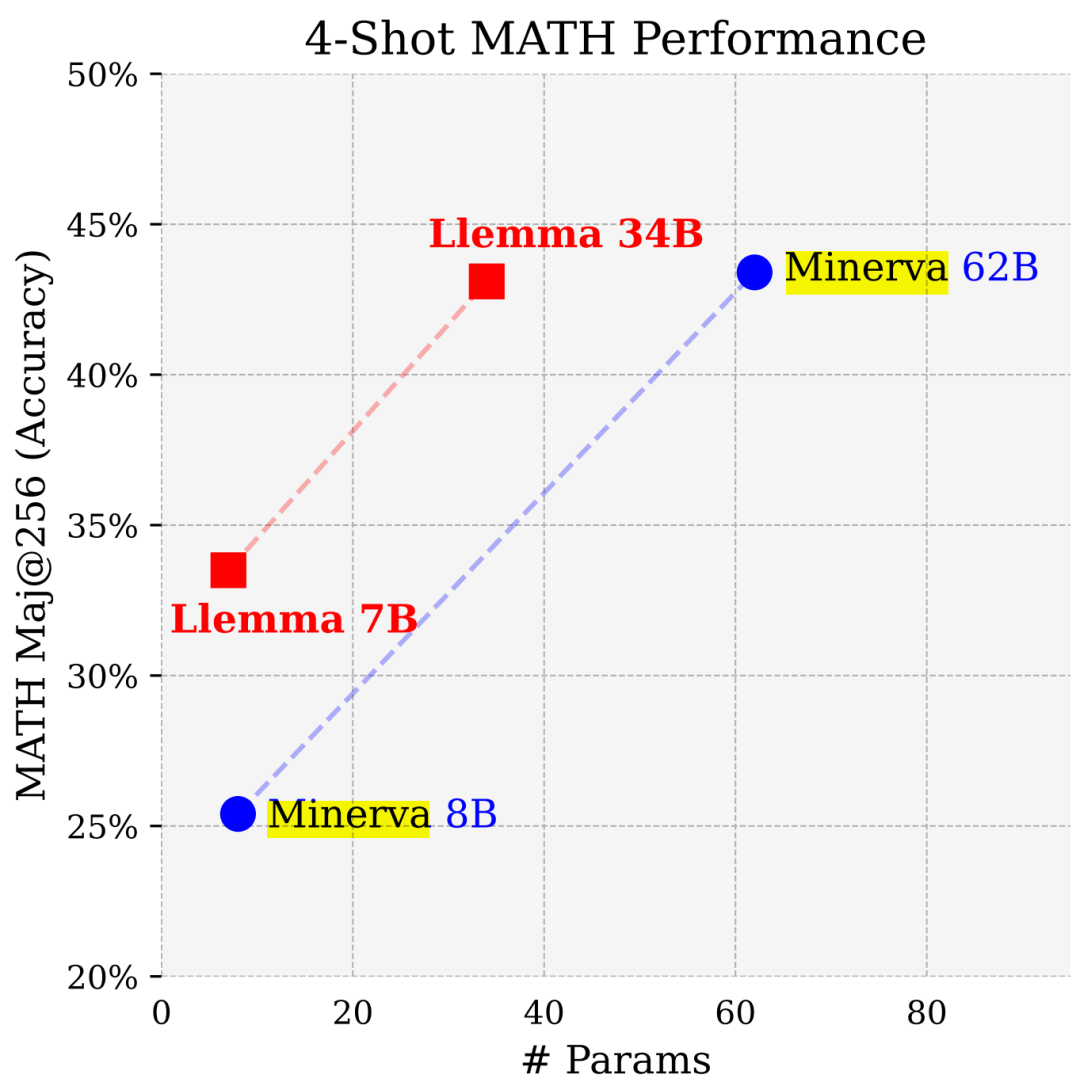
To solve the difficulty of reproducing large models and collaborating, this post-95s student team created a domestic AI open source community
Link: https://news.miracleplus.com/share_link/11086
In the era of large models, how to build a convenient platform that can lower the threshold for communication and collaboration has become a major challenge. The traditional software development collaboration methods we are familiar with, such as Git-based code management and version control, may no longer be applicable in scenarios such as AI R&D that rely more on experiments than deterministic processes. Its complex experimental version management and relatively complex High usage and deployment thresholds often hinder communication and collaboration between experts in different fields. The current AI field requires new collaboration models and tools, including more intuitive and easy-to-use version control and collaboration platforms, so that experts with non-technical backgrounds can easily participate in the model development, evaluation and demonstration process. In other words, both scientific researchers and practitioners hope to achieve more efficient and in-depth collaboration based on the sharing of knowledge and technology to promote further development in the field of AI. Against this background, a new AI open source community platform “SwanHub” was born.
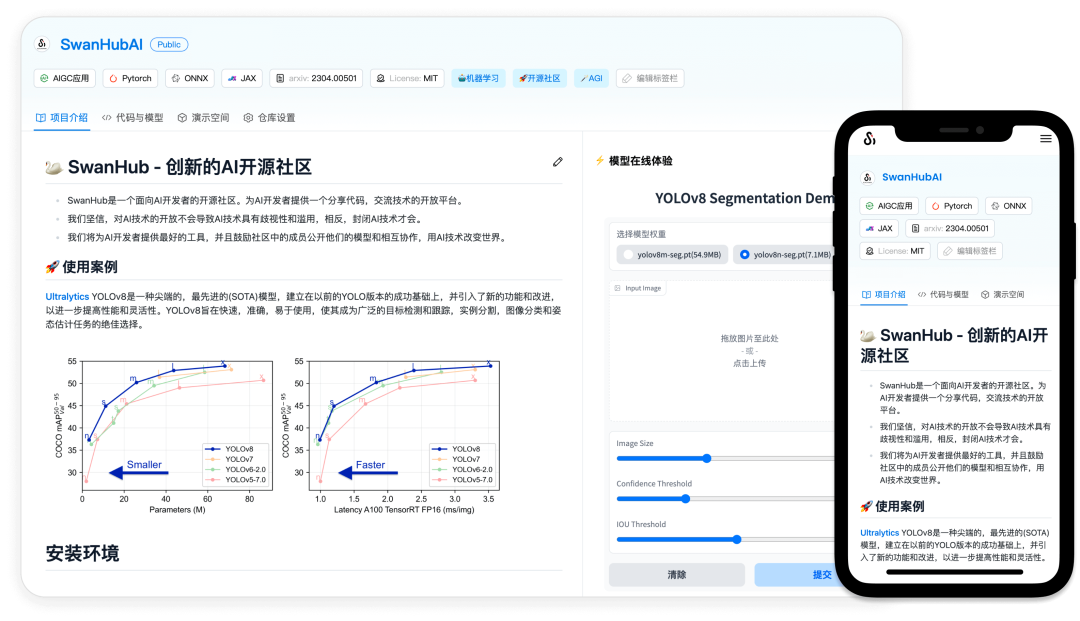
22 billion transistors, IBM machine learning processor NorthPole, energy efficiency increased by 25 times
Link: https://news.miracleplus.com/share_link/11087
As AI systems develop rapidly, their energy requirements are also increasing. Training new systems requires large data sets and processor time, making them extremely energy-intensive. In some cases, smartphones can easily do the job by executing some well-trained systems. However, if it is executed too many times, energy consumption will also increase. Fortunately, there are many ways to reduce the latter’s energy consumption. IBM and Intel have experimented with processors designed to mimic the behavior of actual neurons. IBM also tested performing neural network calculations in phase-change memory to avoid repeated accesses to RAM. Now, IBM has another approach. The company’s new NorthPole processor synthesizes some of the ideas from the above approaches and combines them with a very streamlined way to run computations, creating an energy-efficient chip that can efficiently execute inference-based neural networks. The chip is 35 times more efficient than a GPU in areas such as image classification or audio transcription.
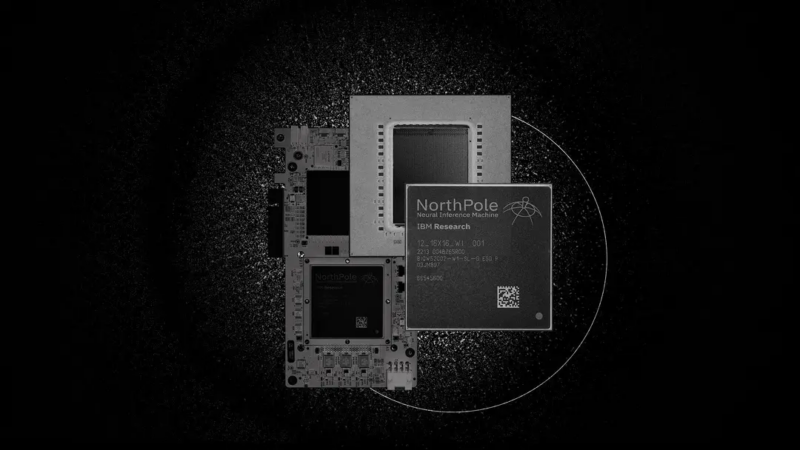
Musk’s first xAI research results released! Co-authored by founding member Yang Ge and Yao class alumni
Link: https://news.miracleplus.com/share_link/11088
Musk’s xAI, the first public research results are here! One of the co-authors is Greg Yang, a founding member of xAI and a disciple of Shing-Tung Yau. Previously, Yang Ge has publicly stated that his research direction in xAI is “Math for AI” and “AI for Math”. One of the key points is to continue his previous research: Tensor Programs, a unified programming language for describing neural network architecture – related results have been applied in GPT-4. This new paper belongs to this series and focuses on “how to train infinitely deep networks”.
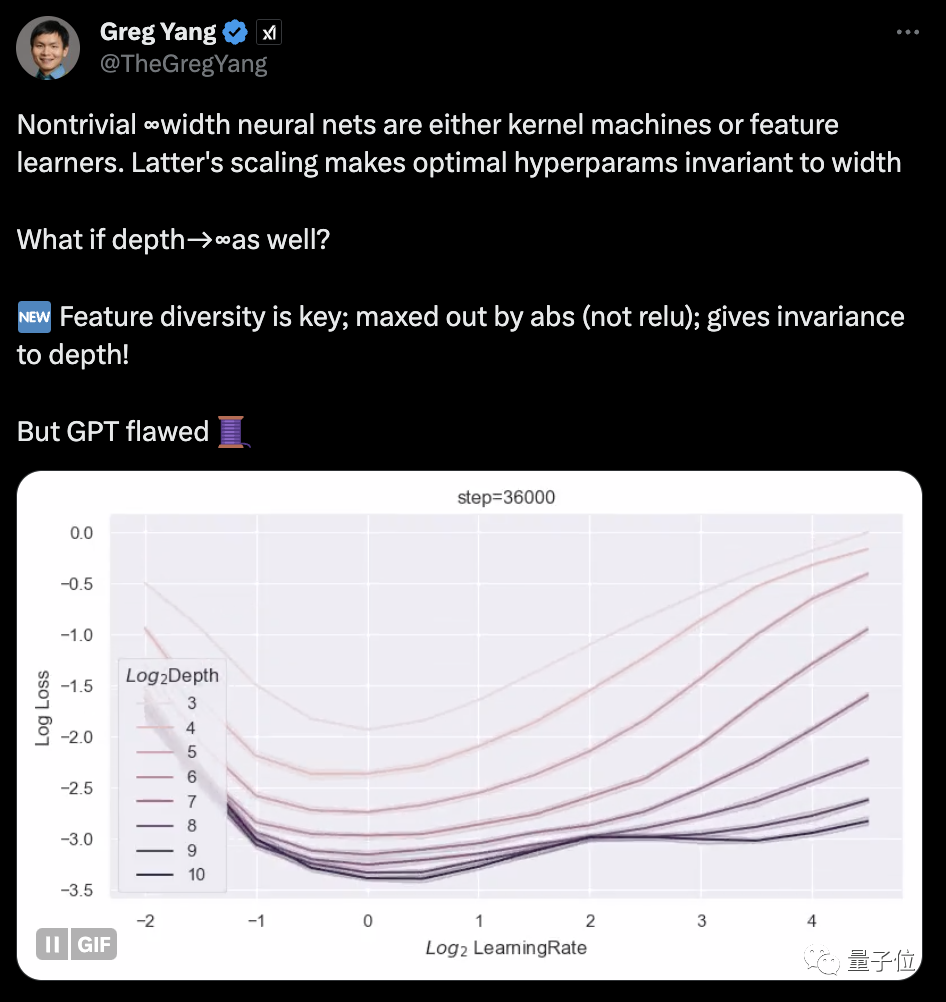
The LLaMA2 context length skyrockets to 1 million tokens, and only one hyperparameter needs to be adjusted | Produced by Qiu Xipeng’s team at Fudan University
Link: https://news.miracleplus.com/share_link/11089
With just a few tweaks, the support context size for large models can be extended from 16,000 tokens to 1 million? ! Still on LLaMA 2, which has only 7 billion parameters. You know, even the most popular Claude 2 and GPT-4 support context lengths of only 100,000 and 32,000. Beyond this range, large models will start to talk nonsense and be unable to remember things. Now, a new study from Fudan University and Shanghai Artificial Intelligence Laboratory has not only found a way to increase the length of the context window for a series of large models, but also discovered the rules.
One line of code improves the performance of large models by 10%, developers: free lunch
Link: https://news.miracleplus.com/share_link/11090
There is a “free lunch” in fine-tuning large models. Just one line of code can improve performance by at least 10%. On Llama 2 with 7B parameters, the performance even doubled, and Mistral also increased by a quarter. Although this method is used in the supervised fine-tuning phase, RLHF models can also benefit from it. Researchers from the University of Maryland, New York University and other institutions proposed a fine-tuning method called NEFT(une). This is a new regularization technique that can be used to improve the performance of supervised fine-tuning (SFT) models. This method has been included in the TRL library by HuggingFace and can be called by adding one more line of code to the import. NEFT is not only easy to operate, but also has no significant cost increase. The author said it looks like a “free lunch”.
Let’s look at pictures of large models more effectively than typing! New research in NeurIPS 2023 proposes a multi-modal query method, increasing the accuracy by 7.8%
Link: https://news.miracleplus.com/share_link/11091
The ability to “read pictures” of large models is so strong, why do they still find the wrong things? For example, confusing bats with bats that don’t look alike, or failing to recognize rare fish in some data sets… This is because when we ask large models to “find things”, the input is often text. If the description is ambiguous or too specific, such as “bat” (bat or bat?) or “demon fish” (Cyprinodon diabolis), the AI will be greatly confused. This leads to the fact that when using large models for target detection, especially in open world (unknown scenes) target detection tasks, the results are often not as good as expected. Now, a paper included in NeurIPS 2023 finally solves this problem. The paper proposes a target detection method MQ-Det based on multi-modal query. It only needs to add an image example to the input to greatly improve the accuracy of large models in finding things. On the benchmark detection data set LVIS, without the need for downstream task model fine-tuning, MQ-Det improves the accuracy of the mainstream detection large model GLIP by about 7.8% on average, and improves the accuracy by an average of 6.3% on 13 benchmark small sample downstream tasks.
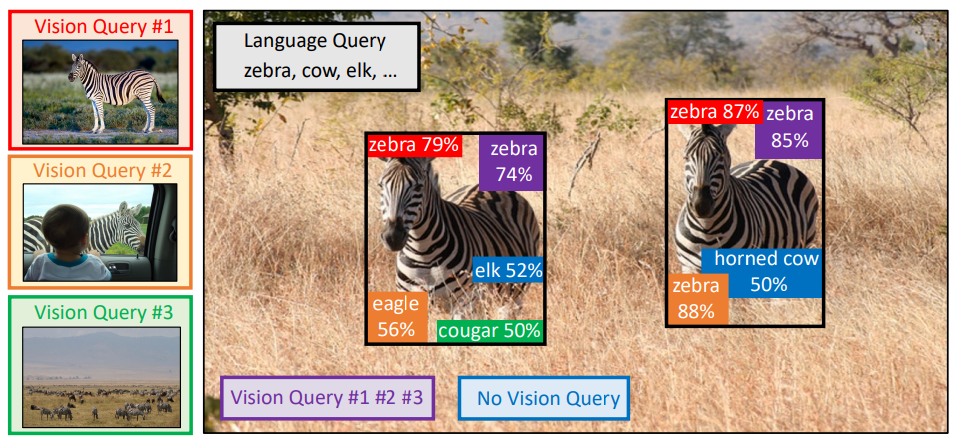