Collection of Large Model Daily on March 8th
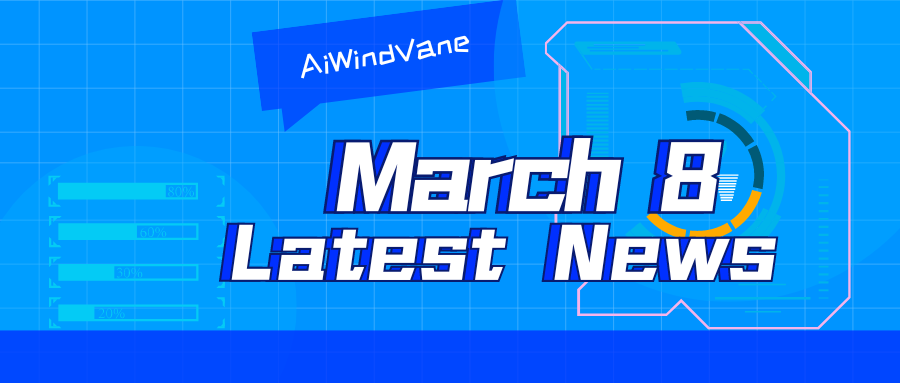
[Collection of Large Model Daily on March 8th] Tsinghua Yao Class undergraduates published two works in a row, the biggest improvement in ten years: matrix multiplication is close to the theoretical optimal; new work by Tian Yuandong and others: breaking through the memory bottleneck, allowing a 4090 pre-trained 7B large model; Hugging Face launches open source robotics project, led by former Tesla scientist
Tsinghua Yao class undergraduates published two works in a row, the biggest improvement in ten years: matrix multiplication is close to the theoretical optimal
Link: https://news.miracleplus.com/share_link/20583
Matrix multiplication, as the basic operation of many GPU operators, is one of the important issues in high-performance computing and the cornerstone of applications such as AI. Its algorithm mechanism itself is quite simple, but in order to achieve faster speeds, people have worked tirelessly for many years, but the degree of optimization has been limited. Today, in a report in “Quantum Magazine”, we saw two papers that further improved the speed of matrix multiplication. Among them, a senior undergraduate student from Yao Class at Tsinghua University participated in the writing of the two papers, which is a pioneer in the field. Algorithmic improvements bring new hope.
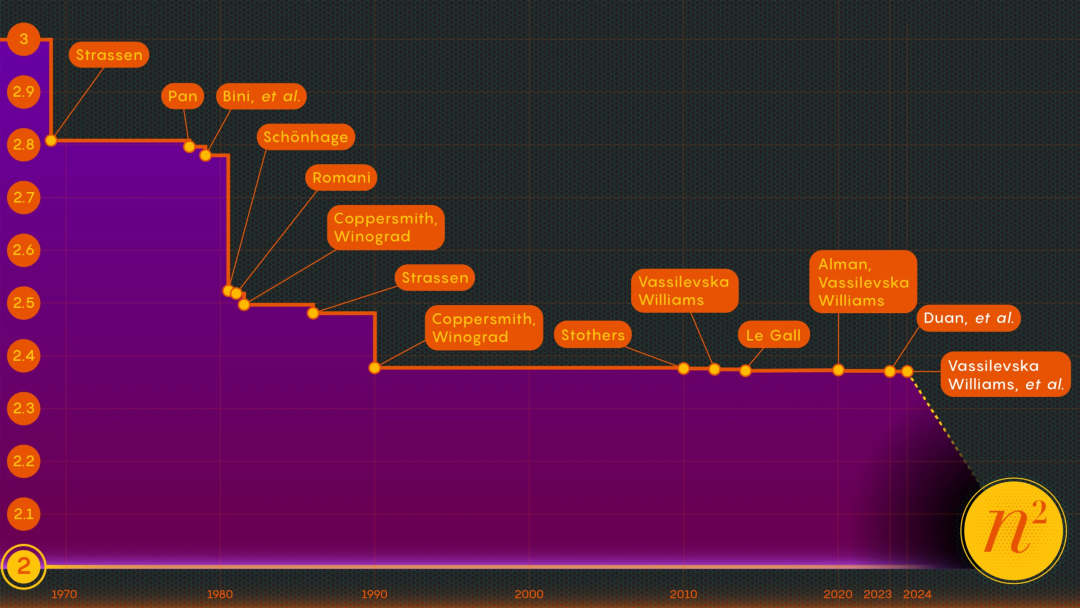
New work by Tian Yuandong and others: Breaking through the memory bottleneck and allowing a 4090 pre-trained 7B large model
Link: https://news.miracleplus.com/share_link/20584
Last month, Meta FAIR Tian Yuandong participated in a study that was well received. In the paper “MobileLLM: Optimizing Sub-billion Parameter Language Models for On-Device Use Cases, they began to roll out small models with less than 1 billion parameters, focusing on mobile devices. Run LLM. On March 6, Tian Yuandong released another study. This time, they focused on LLM memory efficiency. In addition to Tian Yuandong himself, there are also researchers from the California Institute of Technology, the University of Texas at Austin, and CMU. They collaborated to propose GaLore (Gradient Low-Rank Projection), a training strategy that allows full parameter learning but is more memory efficient than common low-rank adaptive methods such as LoRA. This study is the first to demonstrate the feasibility of pre-training a 7B model on a consumer-grade GPU with 24GB of memory (e.g., NVIDIA RTX 4090) without the need for model parallelism, checkpointing, or offloading strategies.
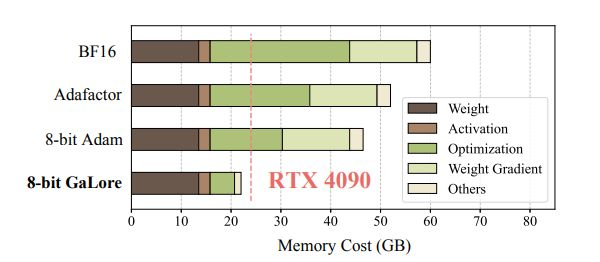
“AI Perspective Eye”, three-time Marr Prize winner Andrew leads a team to solve the problem of occlusion and completion of any object
Link: https://news.miracleplus.com/share_link/20585
Occlusion is one of the most basic but still unsolved problems in computer vision, because occlusion means the lack of visual information, while machine vision systems rely on visual information for perception and understanding, and in the real world, objects block each other. everywhere. The latest work of Andrew Zisserman’s team at the VGG Laboratory at the University of Oxford systematically solved the problem of occlusion completion of arbitrary objects and proposed a new and more accurate evaluation data set for this problem. This work has been praised by MPI boss Michael Black, the official account of CVPR, the official account of the Department of Computer Science of the University of Southern California, etc. on the X platform. The following is the main content of the paper “Amodal Ground Truth and Completion in the Wild”.
Predict all biomolecules, David Baker team’s new protein design tool RoseTTAFold All-Atom in Science
Link: https://news.miracleplus.com/share_link/20586
In the field of proteins, David Baker’s team at the University of Washington has brought new progress. Proteins are indispensable molecules for life, but they are not the only molecules in cells. They must cooperate with other molecules to participate in life processes. In recent years, protein structure prediction algorithms such as AlphaFold and RoseTTAFold have taken the field of structural biology by storm. Deep learning methods have revolutionized the way protein structure is predicted and designed, but are currently limited to pure protein systems. The problem is, these models ignore many types of chemistry that affect protein structure. “For example, a lot of biology involves proteins interacting with small molecules,” said David Baker, a professor at the University of Washington. “This is a hypothesis we wanted to test: Is it possible to train a model that can represent all these different types of molecules?” said Rohith Krishna, first author on the paper. Based on this, Baker’s team developed RoseTTAFold All-Atom (RFAA), which can combine residue-based representations of amino acids and DNA bases with atomic representations of all other groups to analyze proteins, nucleic acids, small molecules, Metals and covalently modified components of a given sequence and chemical structure are modeled.
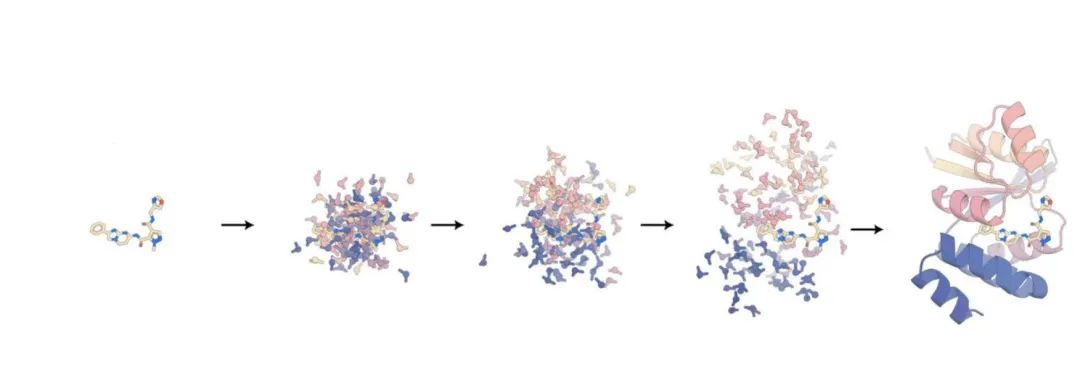
Hugging Face launches open source robotics project, led by former Tesla scientist
Link: https://news.miracleplus.com/share_link/20587
Machine learning and AI model community Hugging Face has launched a new robotics project under the leadership of former Tesla scientist Remi Cadene. Cadene posted the news on X and said that the Hugging Face robotics project will be “open source, not like OpenAl That way”
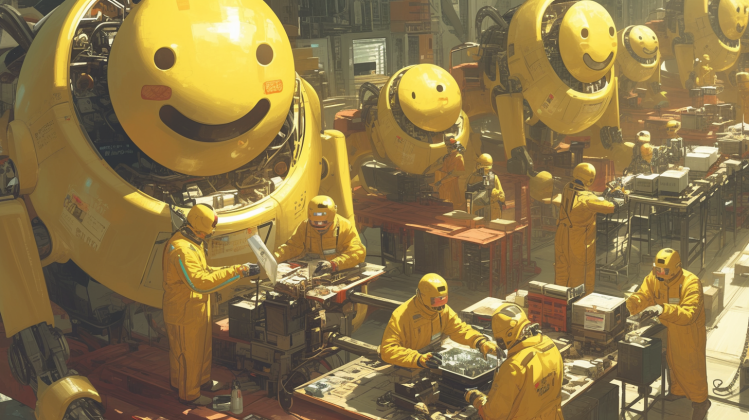
Sources say Samsung is poaching TSMC customers and is expected to win Meta Al chip foundry orders
Link: https://news.miracleplus.com/share_link/20588
As Samsung’s wafer foundry business closely follows TSMC, there are reports that Samsung is poaching TSMC customers and is expected to get Meta’s next-generation self-developed AI chip foundry orders, which will be produced using a 2nm process and become Samsung’s first 2nm customer. Currently, two of Meta’s AI chips are produced by TSMC. Industry insiders analyze that the biggest problem in Samsung’s wafer foundry business is the yield rate. Previously, due to poor yield rates, Apple, Qualcomm and Google have switched to TSMC to place orders. If Samsung OEMs Meta’s next-generation AI chips. The key to whether the cooperation between the two parties is smooth lies in the yield rate.
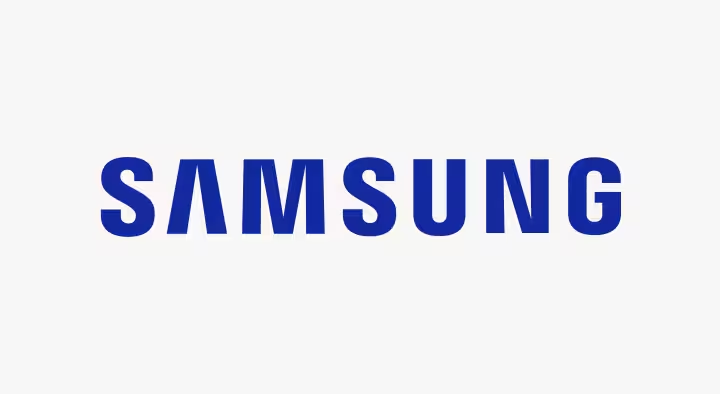