December 29th Large Model Daily Collection
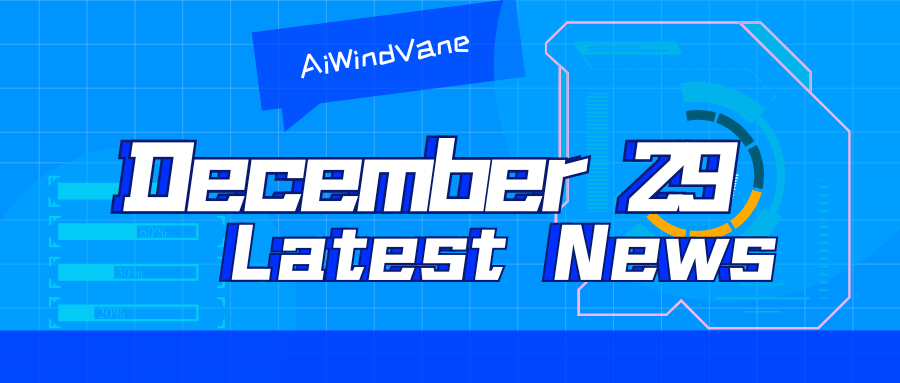
[December 29th Large Model Daily Collection] Using diffusion model to supervise NeRF, Tsinghua Vincent 3D new method becomes new SOTA
New breakthrough in deep network data encoding, Shanghai Jiao Tong University SPARK attended the top computer architecture conference
Link: https://news.miracleplus.com/share_link/14595
As deep neural network (DNNs) models rapidly grow in size and complexity, traditional neural network processing methods are facing severe challenges. Existing neural network compression technology is inefficient when processing neural network models with large parameter scales and high accuracy requirements, and cannot meet the needs of existing applications. Numerical quantization is an effective means of neural network model compression. During the model inference process, the access and calculation of low-width (bit) data can significantly save storage space, memory access bandwidth, and computing load, thereby reducing inference latency and energy consumption. Currently, the bit width of most quantization techniques is 8 bits. More radical quantization algorithms must modify the operating granularity and data flow characteristics of the hardware in order to obtain close to theoretical benefits in real reasoning. Such as mixed precision quantization, activation data quantization and other solutions. On the one hand, these solutions will explicitly increase book-keeping storage overhead and hardware logic, causing actual benefits to decrease [1, 2, 3]. On the other hand, some solutions use distribution characteristics to constrain the quantization range and granularity to reduce the above hardware overhead [4,5]. However, its accuracy loss is also affected by different models and parameter distributions, and it cannot meet the needs of existing applications. To this end, the researchers of this article proposed SPARK technology, a software and hardware co-design for scalable fine-grained mixed-precision coding.
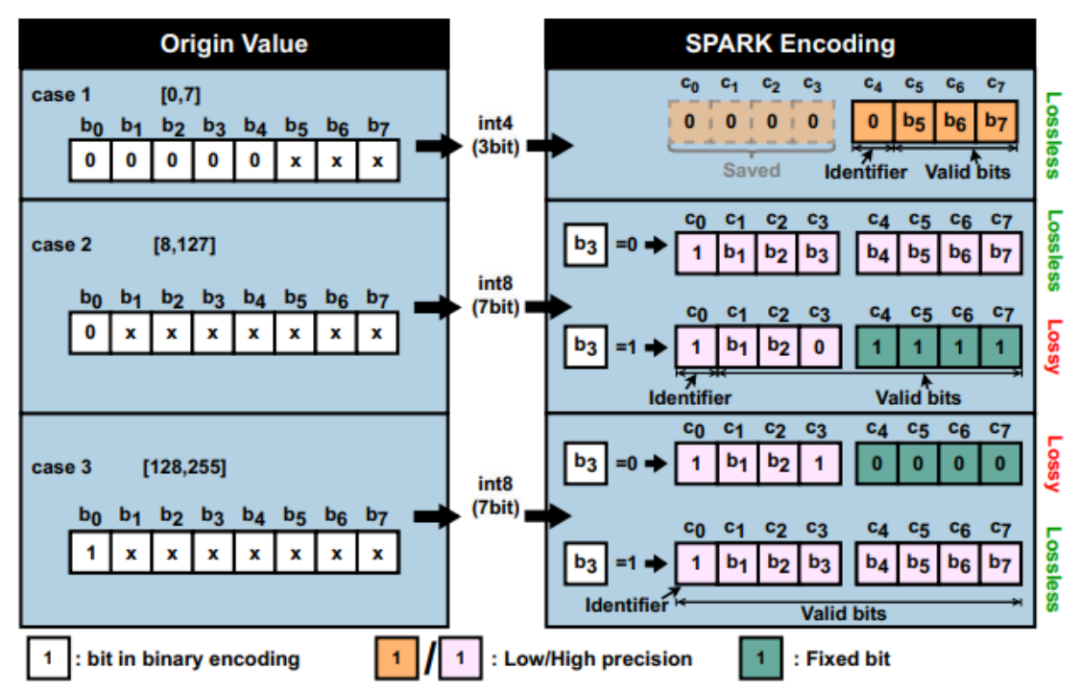
Using diffusion model to supervise NeRF, Tsinghua Vincent 3D new method becomes new SOTA
Link: https://news.miracleplus.com/share_link/14596
The AI model that uses text to synthesize 3D graphics has a new SOTA! Recently, the research group of Professor Liu Yongjin of Tsinghua University proposed a new method of creating 3D images based on diffusion model. Both the consistency between different viewing angles and the matching with prompt words have been greatly improved compared to before. Vincent 3D is a hot research content of 3D AIGC and has received widespread attention from academia and industry. The new model proposed by Professor Liu Yongjin’s research group is called TICD (Text-Image Conditioned Diffusion), which has reached the SOTA level on the T3Bench data set. At present, relevant papers have been published and the code will be open source soon.
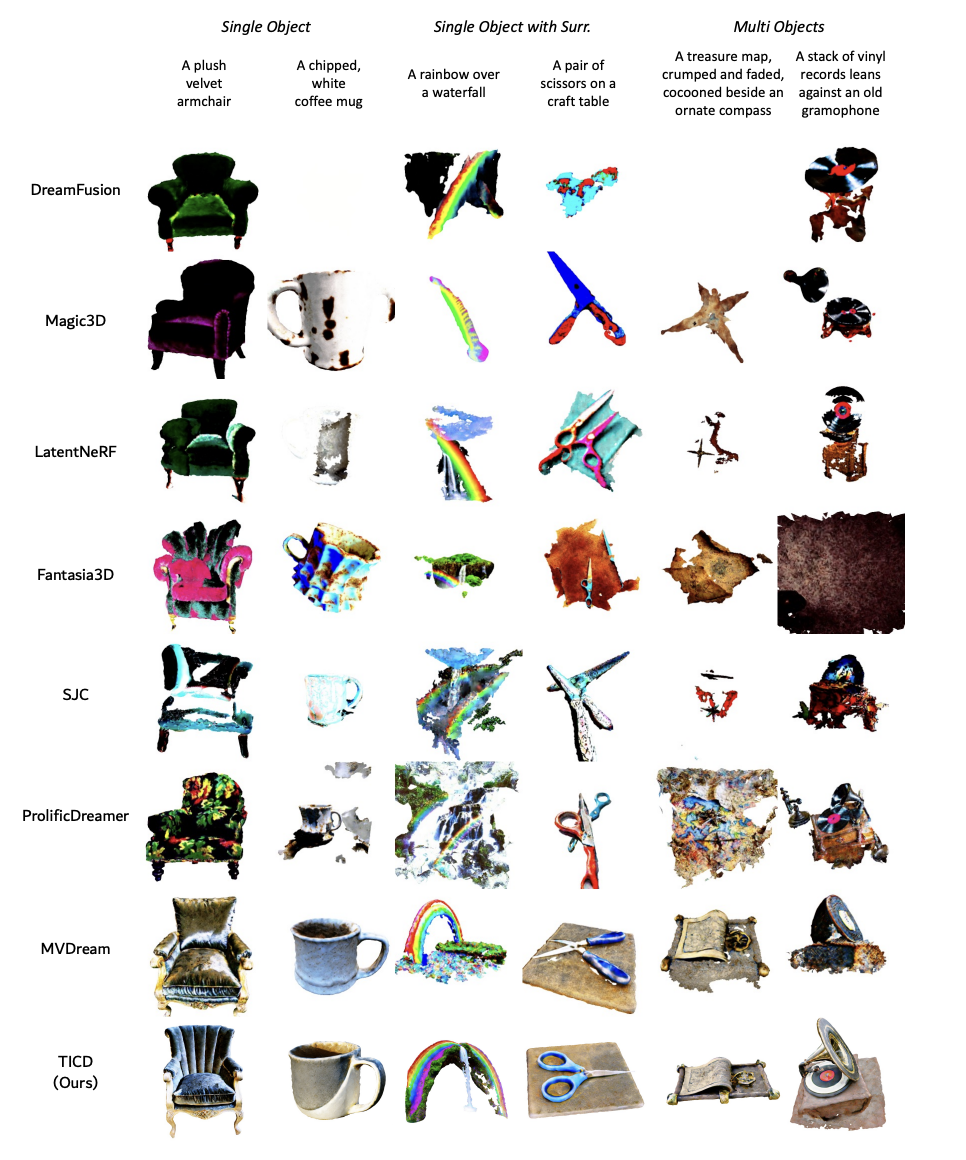
The Shanghai Jiao Tong University & Sun Yat-sen University team used ESMFold, pre-trained language model and Graph Transformer to predict protein binding sites
Link: https://news.miracleplus.com/share_link/14597
Identifying functional sites on proteins, such as binding sites for proteins, peptides, or other biological components, is critical for understanding related biological processes and drug design. However, existing sequence-based methods have limited prediction accuracy because they only consider sequence-adjacent contextual features and lack structural information. Researchers from Shanghai Jiao Tong University and Sun Yat-sen University proposed DeepProSite to identify protein binding sites using protein structure and sequence information. DeepProSite first generates protein structures from ESMFold and sequence representations from pretrained language models. It then uses the Graph Transformer and formulates the binding site predictions into graph node classifications. DeepProSite outperforms current sequence- and structure-based methods on most metrics when predicting protein-protein/peptide binding sites. Furthermore, DeepProSite maintains high performance when predicting unbound structures compared to structure-based prediction methods. DeepProSite can also be extended to the prediction of binding sites for nucleic acids and other ligands, validating its generalization capabilities.
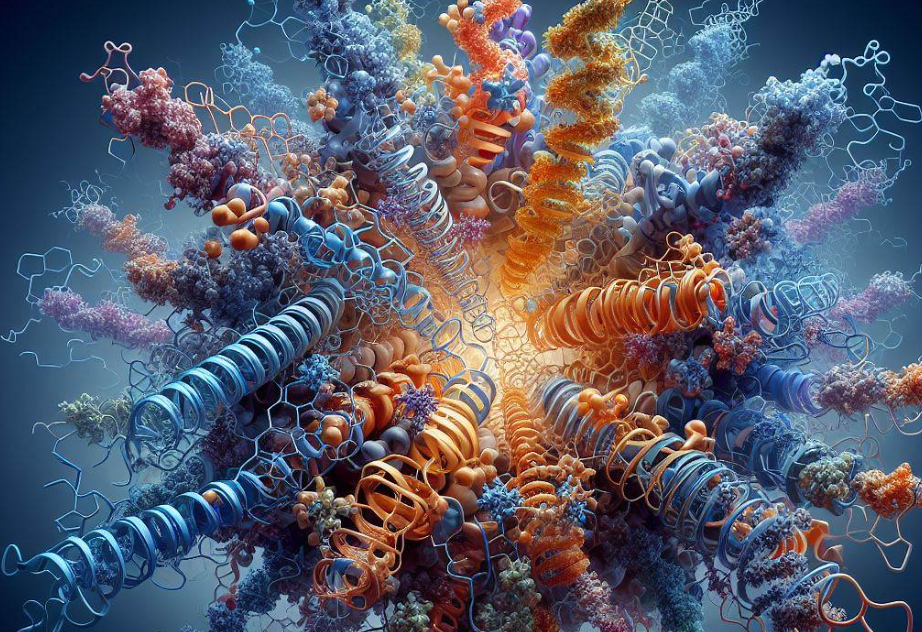