Large Model Daily on February 27
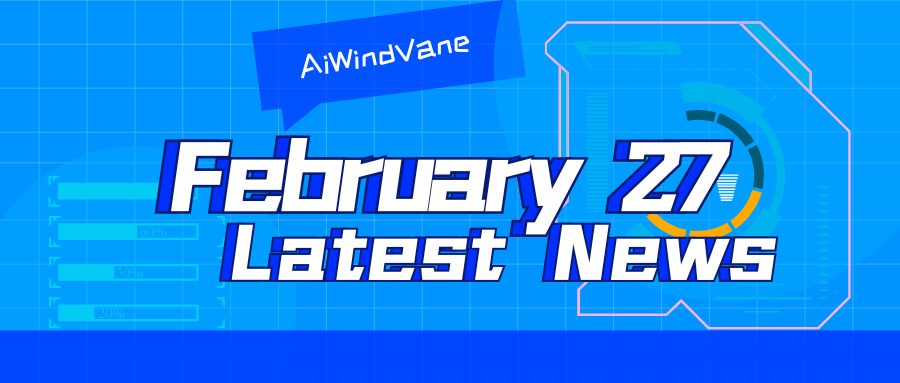
[Large Model Daily on February 27] The end-side is the strongest, Meta Tian Yuandong and others rolled out small models with parameters below 1 billion, LeCun: Tips to start; Large model Scaling Law is also applicable to downstream task performance? The latest research from Stanford and Google is revealed; Mistral AI’s new model benchmarks GPT-4, is not open source and cooperates with Microsoft, netizens: Forgot the original intention; Google’s 10M context window is killing RAG? Is Gemini underrated after being stolen away from the limelight by Sora?
The end-side is the strongest, Meta Tian Yuandong and others rolled out a small model with less than 1 billion parameters, LeCun: Tips to start
Link: https://news.miracleplus.com/share_link/19630
“Running LLM on mobile devices? It may require some skills from Meta.” Just now, Turing Award winner Yann LeCun said on his personal social platform. The research he promoted comes from the latest Meta paper “MobileLLM: Optimizing Sub-billion Parameter Language Models for On-Device Use Cases”. Among the many authors, we are also familiar with Tian Yuandong from Meta FAIR. Tian Yuandong said: “The performance of our MobileLLM pre-trained model (125M/350M) reaches SoTA, especially in chat/API calls. In addition, an interesting research in this work is weight sharing across Transformer layers, which not only It saves parameters and reduces delays in the inference process.”
MATRIX: Social simulation promotes self-alignment of large model values and is more “considerate” than GPT4
Link: https://news.miracleplus.com/share_link/19631
Models such as ChatGPT rely on reinforcement learning based on human feedback (RLHF), which proposes a solution by encouraging annotators’ preferred responses and penalizing unpopular feedback. However, RLHF faces problems such as high cost, difficulty in optimization, and inability to perform in the face of super-human level models. To reduce or even eliminate reliance on human supervision, Anthropic launched Constitutional AI, which is designed to require language models to follow a set of human rules when answering. At the same time, OpenAI’s research provides a new perspective on the alignment of super-human-level models by using weak models to supervise strong models. Nevertheless, due to the ever-changing instructions given by users, applying a fixed set of social rules to LLMs is not flexible enough; moreover, the supervisory improvement effect of weak models on strong models is not yet obvious. In order to solve the challenge of value alignment of these large language models, the scientific research team of Shanghai Jiao Tong University and Shanghai Artificial Intelligence Laboratory () published a new work “Self-Alignment of Large Language Models via Monopolylogue-based Social Scene Simulation”, which proposed a Original self-alignment strategy – social scene simulation. The core idea of this approach is that the formation and development of human social values originate from the interaction and social influence among all participants in society. Applying the analogy to LLMs, by simulating the social scenarios involved in user instructions and LLMs answers, the model is able to observe the possible social impact of its answers, thereby better understanding the social harm that the answers may bring.
Does Large Model Scaling Law also apply to downstream task performance? The latest research from Stanford and Google reveals the secrets
Link: https://news.miracleplus.com/share_link/19632
The success of large models is largely due to the existence of Scaling Law. This law quantifies the relationship between model performance and design factors such as training data size and model architecture, and provides the basis for model development, resource allocation and selection of appropriate training. Data provides valuable guidance. A large number of previous studies have focused on the scaling law of upstream complexity or cross-entropy loss (i.e., evaluation on pre-training data), but in practical applications, the model usually undergoes a transfer learning process: first, it is performed on unsupervised data. Pre-train and then fine-tune for specific downstream tasks such as encoding or translation. So, can Scaling Law be used to predict downstream task performance? This critical question remains largely unanswered. In a recent work, researchers from Stanford University and Google explored the Scaling Law of transfer learning.
The University of Cambridge team uses deep learning tools to evaluate the naturalness of nanobodies to help develop antibody drugs
Link: https://news.miracleplus.com/share_link/19633
Monoclonal antibodies have emerged as key treatments. In particular, nanobodies (small, single-domain antibodies expressed naturally in camelids) quickly gained momentum after the approval of the first nanobody drug in 2019. Nonetheless, the development of these biologics as therapeutic agents remains a challenge. Although in vitro directed evolution techniques are well-established and relatively fast and cheap to deploy, the gold standard for generating therapeutic antibodies remains discovery from animal immunizations or patients. Immune system-derived antibodies tend to have favorable properties in vivo, including long half-life, low reactivity with self-antigens, and low toxicity. In the latest study, researchers at the University of Cambridge have unveiled AbNatiV, a deep learning tool for assessing the nativity of antibodies and nanobodies, i.e. their likelihood of belonging to the immune system-derived distribution of human antibodies or camel nanobodies. AbNatiV is a versatile tool that can accurately predict the nativeness of Fv sequences from any source, including synthetic libraries and computational designs. It provides an interpretable score that can predict the likelihood of immunogenicity, and a residue-level profile that can guide the engineering of antibodies and nanobodies that are indistinguishable from immune system-derived antibodies and nanobodies. The team further introduced an automated humanization process and applied it to two nanobodies. Laboratory experiments show that, unlike Nanobodies that are humanized using traditional structure and residue frequency analysis, AbNatiV humanized Nanobodies retain binding and stability that is comparable to or better than that of wild type.
Mistral AI’s new model benchmarks against GPT-4. It is not open source and cooperates with Microsoft. Netizens: Forgot the original intention
Link: https://news.miracleplus.com/share_link/19634
In the field of generative AI, another heavyweight product has appeared. On Monday night, Mistral AI officially released the “flagship” large model Mistral Large. Different from the previous series of models, the version released by Mistral AI this time has stronger performance and larger size, directly benchmarking OpenAI’s GPT-4. The emergence of new models is also accompanied by a transformation in the company’s general direction. With the launch of Mistral Large, Mistral AI launched a chat assistant called Le Chat (compared to ChatGPT), and anyone can try the effect.
NVIDIA new graphics card released! Notebook AI drawing speed is 14 times faster, and the thin and light notebook can also be used as an AI workstation
Link: https://news.miracleplus.com/share_link/19635
Academician Huang’s new nuclear bomb is here! A new consumer-grade graphics card designed to speed up notebook large-model applications. Just over the past two days at MWC, NVIDIA has launched a new GPU-RTX 500 and RTX 1000. Compared to using only the CPU, the new RTX 500 can provide up to 14 times the generative AI performance for models such as Stable Diffusion! Not only that, with RTX 500, the speed of photo editing using AI will also be increased by 3 times, and the performance of 3D rendering graphics will be increased by 10 times. More importantly, RTX 500 and RTX 1000 are workstation graphics cards for thin and light laptops and belong to Nvidia’s Ada Generation series. Even with such improvements in performance, Nvidia still positions the two at the “entry-level” level, focusing on making ordinary notebooks have powerful AI capabilities.
DeepMind CEO’s latest New York Times interview: AGI will make energy cheap or even free, and the nature of money will also change
Link: https://news.miracleplus.com/share_link/19636
Google DeepMind CEO Demis Hassabis recently joined The New York Times for a conversation. Demis talked about Google’s latest AI breakthroughs, building AGI, and what will happen in a world where computers can do every job? Additionally, Demis says AI-designed drugs and treatments that can cure truly horrific diseases are only a few years away. He believed that energy became free or cheap, leading to a change in the nature of money.
Google’s 10M context window is killing RAG? Is Gemini underrated after being stolen away from the limelight by Sora?
Link: https://news.miracleplus.com/share_link/19637
Google is definitely one of the most depressing companies recently: its own Gemini 1.5 has just been released, and it has been stolen by OpenAI’s Sora, which can be called the “Wang Feng” in the AI industry. Specifically, Google is launching Gemini 1.5 Pro, the first version of Gemini 1.5 for early testing. It is a medium-sized multimodal model (across text, video, audio) with similar performance levels to Google’s largest model to date, 1.0 Ultra, and introduces groundbreaking experimental features in long-context understanding. It can stably handle up to 1 million tokens (equivalent to 1 hour of video, 11 hours of audio, more than 30,000 lines of code, or 700,000 words), with a limit of 10 million tokens (equivalent to the “Lord of the Rings” trilogy), Set a record for the longest context window. In addition, it can learn to translate a small language using only a 500-page grammar book, 2,000 bilingual entries, and 400 additional parallel sentences (there is no relevant information on the Internet), and its translation score is close to that of human learners. Many people who have tested the Gemini 1.5 Pro have said that this model is underrated. For example, someone tried to throw the entire code base downloaded from Github along with issues to Gemini 1.5 Pro. As a result, it not only understood the entire code base, but also identified the most urgent issues and fixed them.