February 8-18 Big Model Daily Special
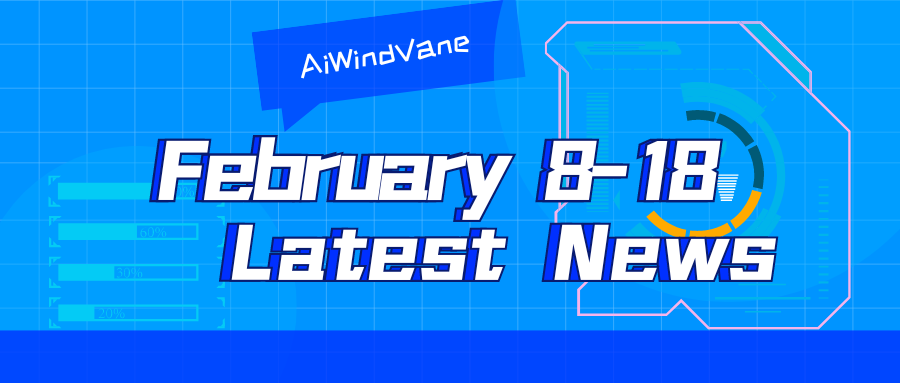
[February 8-18 Big Model Daily Special] Spring Festival gift pack! OpenAI’s first video generation model is released, a 60-second high-definition masterpiece that has impressed netizens; Google Gemini 1.5 is quickly online: MoE architecture, 1 million contexts; sudden! AI guru Andrej Karpathy leaves OpenAI; US$7 trillion: OpenAI’s super-large chip plan is exposed to reshape the global semiconductor industry
New work by Chen Danqi’s team: The amount of data is reduced by 95%, and the performance of large models is even stronger! Less is More
https://news.miracleplus.com/share_link/18484
The cost of building large models has been reduced again! This time, the data volume was reduced by 95%. Chen Danqi’s team recently proposed a method to reduce costs for large models – the data selection algorithm LESS, which only selects 5% of the data most relevant to the task for fine-tuning instructions. The effect is better than using the entire data set. Instruction fine-tuning is a key step in making the basic model become a ChatGPT assistant model.
Stanford’s most powerful housework robot ALOHA 2 is here, costing less than 200,000, teamed up with Google DeepMind, completely open source
https://news.miracleplus.com/share_link/18485
In 2023, Stanford University and other institutions launched ALOHA, a low-cost open source hardware system for remote operation of dual manipulators. It can remotely operate and complete complex and rich tasks such as assembling chains and lifting table tennis balls. In January this year, Google DeepMind and Stanford jointly launched Mobile ALOHA, which can also perform remote operations and imitate two-hand operations, and achieve remote operations in a large space through a mobile base. In this way, it is proficient in preparing vegetables, stir-frying, cooking, washing, playing with cats, and watering flowers. It is a veritable household robot that has become popular. Today, Google DeepMind and Stanford launched an enhanced version of ALOHA – ALOHA 2. Compared with the first generation, ALOHA 2 has stronger performance, ergonomic design and robustness, and costs less than 200,000 yuan.
MIT and IBM teams use clever AI methods to solve “brute force” math problems
https://news.miracleplus.com/share_link/18486
Since the time of Newton, the fundamental laws of nature—optics, acoustics, engineering, electronics—have ultimately been boiled down to a set of important, broad equations. Now, researchers have found a new way to use brain-inspired neural networks to solve these equations more efficiently than before, with many potential applications in science and engineering. The relevant research was titled “Physics-enhanced deep surrogates for partial differential equations” and was published in “Nature Machine Intelligence”.
“Smart Emergence” of Speech Generation: 100,000 Hours of Data Training, Amazon Offers 1 Billion Parameters BASE TTS
https://news.miracleplus.com/share_link/18487
With the rapid development of generative deep learning models, natural language processing (NLP) and computer vision (CV) have undergone a fundamental transformation from specialized models with supervised training to those that can be completed with limited explicit instructions. A general model for various tasks. This shift is also taking place in the fields of speech processing and text-to-speech (TTS), where models are able to leverage thousands of hours of data to make synthesis results closer and closer to human-like speech. In a recent study, Amazon officially launched BASE TTS, increasing the parameter size of the TTS model to an unprecedented level of 1 billion.
Fudan TravelPlanner lets large language models challenge journey planning
https://news.miracleplus.com/share_link/18488
In the development process of artificial intelligence, planning has always been one of the core pursuits. However, early AI agents mainly focused on constrained environments due to the lack of diverse cognitive foundations required for human-level planning. With the emergence of large language models (LLMs), a new generation of language agents has demonstrated interesting capabilities such as tool use and reasoning. This raises the question: Will these language agents be able to plan in more complex environments that were beyond the reach of previous AI agents? To explore this issue in depth, we propose a new planning benchmark, TravelPlanner, that focuses on a common real-world planning scenario: travel planning. This is a challenging task even for humans, but one that most people can successfully complete given the proper tools and enough time.
Huawei’s large Pangu model becomes “small”, and it can also be played at 1.5B
https://news.miracleplus.com/share_link/18489
The emergence of a series of models such as ChatGPT has attracted global attention with its powerful performance. It is expected to change the interaction between humans and computers and be applied to thousands of industries. However, these large models actually require extremely high memory and computing resources, which limits their application in various scenarios. For example, GPT-3 with 175B parameters requires approximately 700GB of memory when stored using the FP32 data type. Although the 7B parameter model is relatively more efficient, its resource requirements still make it difficult to directly deploy on edge devices such as mobile phones. Furthermore, although many studies have successfully built multiple large language models that perform well, they often adopt similar training strategies. On the one hand, a large amount of work focuses on collecting and cleaning data, with less emphasis on studying effective training strategies. On the other hand, the training of large models requires extremely high investment in computing resources, making it impractical to explore a large number of optimization strategies. In this work, the author uses a 1B language model as a carrier to discuss in detail how small language models should make elixirs. The author conducts research from three perspectives: model structure, parameter initialization, and model optimization methods: and summarizes four alchemy techniques to improve the effect of small language models.
Google proposes a new RLHF method: eliminating reward models and eliminating the need for adversarial training
https://news.miracleplus.com/share_link/18490
The success of large language models (LLM) is inseparable from “reinforcement learning based on human feedback (RLHF)”. RLHF can be roughly divided into two stages. First, given a pair of preferred and unpreferred behaviors, a reward model is trained to assign a higher score to the former by classifying the target. This reward function is then optimized through some kind of reinforcement learning algorithm. However, key elements of the reward model may have some undesirable effects. Researchers from Carnegie Mellon University (CMU) and Google Research jointly proposed a simple, theoretically rigorous, and experimentally effective new RLHF method – Self-Play Preference Optimization (SPO) ). This approach eliminates reward models and does not require adversarial training.
The road to trust in large language models: TrustLLM fully revealed
https://news.miracleplus.com/share_link/18491
The outstanding capabilities of large language models (LLMs) in NLP have attracted widespread attention, affecting applications in all aspects of our lives. The outstanding capabilities of LLMs are attributed to several factors, such as using large-scale raw text from the Web as training data, using transformer architecture design with a large number of parameters, and advanced model training schemes. However, the rise of LLMs has also introduced concerns about their credibility. Unlike traditional language models, LLMs have unique characteristics that may lead to trustworthiness issues. TrustLLM is a unified framework for a comprehensive analysis of the trustworthiness of LLM, including a comprehensive review of existing work, principles of different dimensions of trustworthy LLM, a new test benchmark, and comprehensive trustworthiness of mainstream LLM degree assessment.
ICLR 2024 | The first zero-order optimized deep learning framework, MSU and LLNL propose DeepZero
https://news.miracleplus.com/share_link/18492
Today I introduce an article from Michigan State University and Lawrence Livermore National Laboratory about the zeroth-order optimization deep learning framework “DeepZero: Scaling up Zeroth-Order Optimization for “Deep Model Training”, this article was accepted by ICLR 2024, and the code has been open source.
RAG or fine-tuning? Microsoft has released a guide to the construction process of large model applications in specific fields
https://news.miracleplus.com/share_link/18493
There are generally two common approaches to incorporating proprietary and domain-specific data when building large language model applications: retrieval enhancement generation and fine-tuning. Retrieval enhancement generates hints augmented by external data, while fine-tuning integrates additional knowledge into the model itself. However, the advantages and disadvantages of these two methods are not fully understood. In this article, researchers from Microsoft introduce a new focus: creating AI assistants for an industry (agriculture) that requires specific context and adaptive responses. This paper presents a comprehensive large language model pipeline for generating high-quality, industry-specific questions and answers. The approach involves a systematic process of identifying and collecting relevant documents covering a wide range of agricultural topics. These documents are then cleaned and structured to generate meaningful question-answer pairs using a basic GPT model. The generated question-answer pairs are then evaluated and filtered based on their quality.
Performance improvement and cost reduction, this is the latest research progress of distributed reinforcement learning algorithms
https://news.miracleplus.com/share_link/18494
Deep Reinforcement Learning (DRL) is a recognized and effective technology for solving continuous decision-making problems. In order to deal with the data inefficiency problem of DRL, inspired by distributed machine learning technology, distributed deep reinforcement learning (DDRL) has been proposed and successfully applied in the fields of computer vision and natural language processing. Some people believe that distributed reinforcement learning is the only way for deep reinforcement learning to move toward large-scale applications and solve complex decision-making spaces and long-term planning problems. Distributed reinforcement learning is a comprehensive research subfield that requires mutual awareness and collaboration of deep reinforcement learning algorithms and distributed system design. Taking into account the tremendous progress of DDRL, we have organized a series of articles on the development history, challenges and opportunities of DDRL technology. We reviewed the classic DDRL framework in part 1. In this part, we use three papers to specifically analyze the current life of DDRL — the latest research progress.
Google Gemini 1.5 is launched quickly: MoE architecture, 1 million contexts
https://news.miracleplus.com/share_link/18496
Gemini 1.5 builds on research and engineering innovations in Google’s foundational model development and infrastructure, including a new Mix of Experts (MoE) architecture that makes training and serving Gemini 1.5 more efficient. What Google is now rolling out is the first version of Gemini 1.5 for early testing — Gemini 1.5 Pro. It is a medium-sized multi-modal model optimized for scaling across a variety of tasks, delivering performance levels similar to Google’s largest model to date, 1.0 Ultra, and introducing groundbreaking experimental features in long-context understanding. Gemini 1.5 Pro comes with 128,000 token context windows. But starting today, a handful of developers and enterprise customers can try it out in a contextual window of up to 1 million tokens through a private preview of AI Studio and Vertex AI. Google has also made some optimizations,